API
If you're looking for an API, you can choose from your desired programming language.
1
2
3
4
5
6
7
8
9
10
11
12
13
14
15
16
17
18
19
20
21
22
23
24
25
26
27
28
29
30
31
32
33
34
35
36
37
38
39
40
import requests
import base64
# Use this function to convert an image file from the filesystem to base64
def image_file_to_base64(image_path):
with open(image_path, 'rb') as f:
image_data = f.read()
return base64.b64encode(image_data).decode('utf-8')
# Use this function to fetch an image from a URL and convert it to base64
def image_url_to_base64(image_url):
response = requests.get(image_url)
image_data = response.content
return base64.b64encode(image_data).decode('utf-8')
# Use this function to convert a list of image URLs to base64
def image_urls_to_base64(image_urls):
return [image_url_to_base64(url) for url in image_urls]
api_key = "YOUR_API_KEY"
url = "https://api.segmind.com/v1/sd1.5-deepspacediffusion"
# Request payload
data = {
"prompt": "jwst, nebula, spiral galaxy,mixed colors,outerspace, hyper quality, intricate detail, ultra realistic, maximum detail, foreground focus, instagram, 8k, volumetric light, cinematic, octane render, uplight, no blur, 8k",
"negative_prompt": "ng_deepnegative_v1_75t,badhandv4, (worst quality:2), (low quality:2), (normal quality:2), lowres,watermark, single color, monochrome",
"scheduler": "dpmpp_2m",
"num_inference_steps": 30,
"guidance_scale": 7,
"samples": 1,
"seed": 720692329,
"img_width": 512,
"img_height": 768,
"base64": False
}
headers = {'x-api-key': api_key}
response = requests.post(url, json=data, headers=headers)
print(response.content) # The response is the generated image
Attributes
Prompt to render
Prompts to exclude, eg. 'bad anatomy, bad hands, missing fingers'
Type of scheduler.
Allowed values:
Number of denoising steps.
min : 20,
max : 100
Scale for classifier-free guidance
min : 0.1,
max : 25
Number of samples to generate.
min : 1,
max : 4
Seed for image generation.
Width of the image.
Allowed values:
Height of the Image
Allowed values:
Base64 encoding of the output image.
To keep track of your credit usage, you can inspect the response headers of each API call. The x-remaining-credits property will indicate the number of remaining credits in your account. Ensure you monitor this value to avoid any disruptions in your API usage.
Deep Space Diffusion
Deep Space Diffusion stands out with its specialized training on high-quality space imagery. By incorporating visuals from the James Webb Space Telescope and Judy Schmidt's astrophotography, the model offers unparalleled accuracy and beauty in space-themed image generation. This model is a gateway to creating breathtaking cosmic imagery, perfect for astronomers, artists, and space enthusiasts.
Advantages
-
Specialized Training: Harnesses images from the James Webb Space Telescope and Judy Schmidt for authentic space visuals..
-
Easy-to-Use Token: Simply include "JWST" in your prompts (e.g., "jwst, green spiral galaxy") to activate the model's unique style..
-
Optimized for Creativity:: Ideal for educational content, artistic projects, and space exploration visualizations.
Use Cases
-
Educational Content:Create accurate and engaging visuals for astronomy education and space exploration.
-
Scientific Visualization: Assist researchers and astronomers in visualizing celestial phenomena and theoretical concepts.
-
Artistic Projects: Generate unique space-themed artwork for exhibitions, digital art, or personal collections.
-
Personal Projects: For space enthusiasts and hobbyists looking to create their own interpretations of the cosmos.
Other Popular Models
illusion-diffusion-hq
Monster Labs QrCode ControlNet on top of SD Realistic Vision v5.1
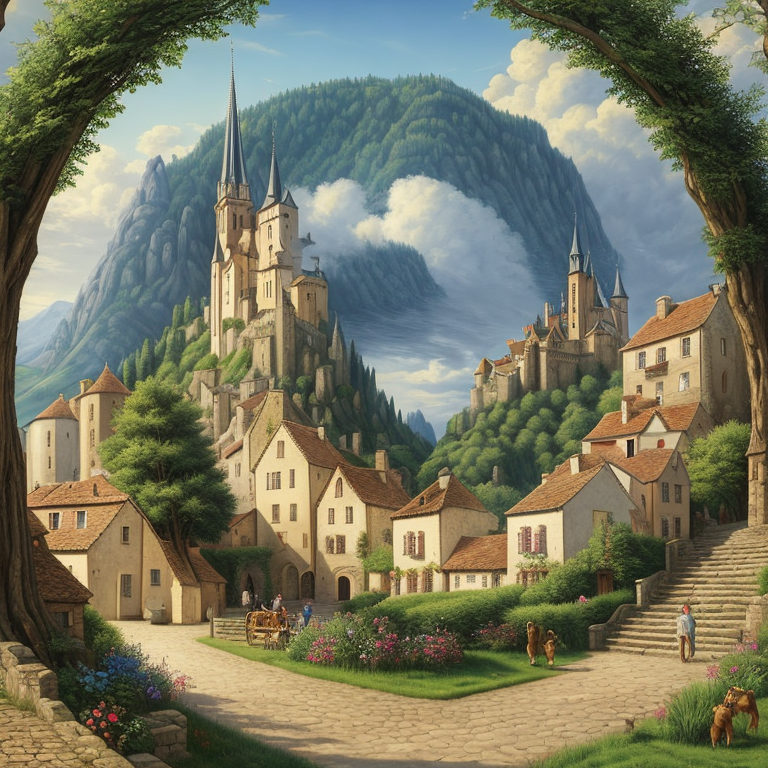
faceswap-v2
Take a picture/gif and replace the face in it with a face of your choice. You only need one image of the desired face. No dataset, no training
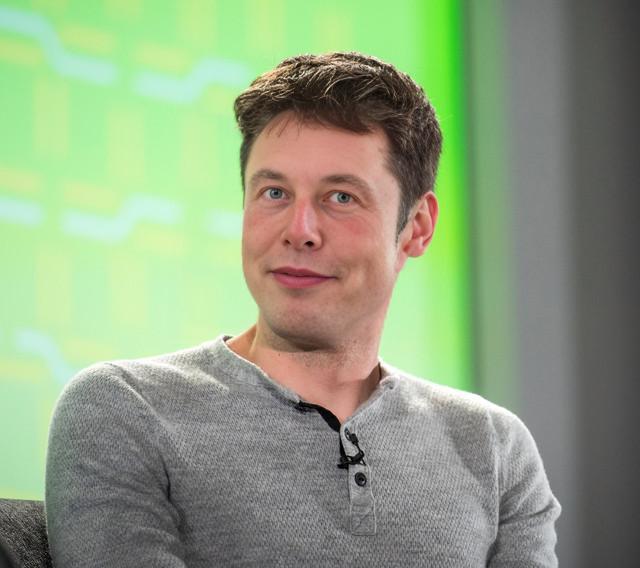
codeformer
CodeFormer is a robust face restoration algorithm for old photos or AI-generated faces.
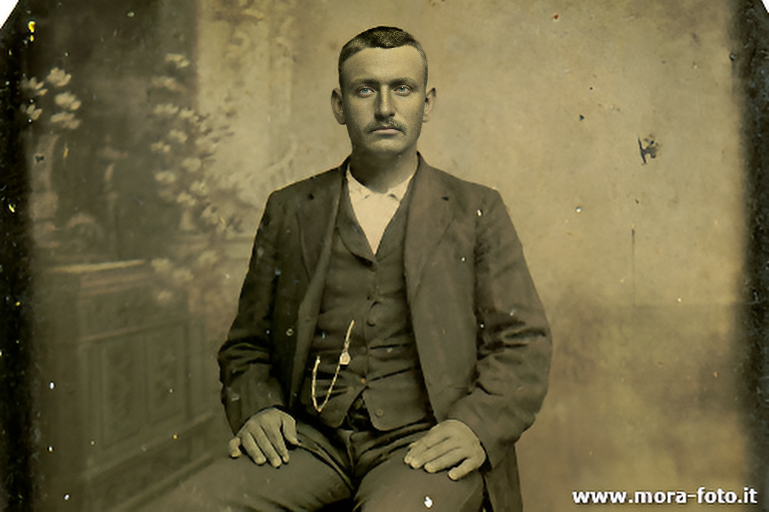
sd2.1-faceswapper
Take a picture/gif and replace the face in it with a face of your choice. You only need one image of the desired face. No dataset, no training
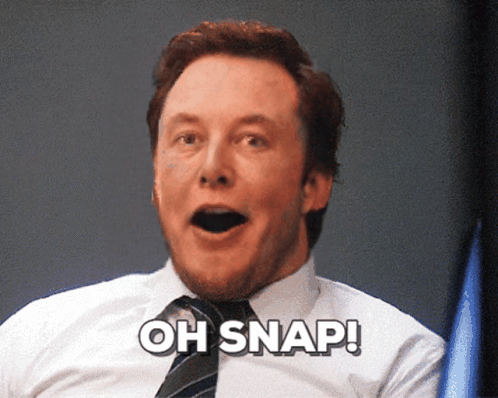