API
If you're looking for an API, you can choose from your desired programming language.
1
2
3
4
5
6
7
8
9
10
11
12
13
14
15
16
17
18
19
20
21
22
23
24
25
26
27
28
29
30
31
32
33
34
35
36
import requests
import base64
# Use this function to convert an image file from the filesystem to base64
def image_file_to_base64(image_path):
with open(image_path, 'rb') as f:
image_data = f.read()
return base64.b64encode(image_data).decode('utf-8')
# Use this function to fetch an image from a URL and convert it to base64
def image_url_to_base64(image_url):
response = requests.get(image_url)
image_data = response.content
return base64.b64encode(image_data).decode('utf-8')
api_key = "YOUR_API_KEY"
url = "https://api.segmind.com/v1/sd1.5-disneyB"
# Request payload
data = {
"prompt": "(8k, best quality, masterpiece:1.2), (finely detailed),kuririn, 1boy,solo,cowboy shot, (bald:1.3), dwarf,(lime green long wizard robes:1.5), smile,open mouth, outdoors, (hut), forest, flowers, parted lips,black eyes,(black belt:1.3), (buckle), lime-green long sleeves,((purple stocking hat:1.2)), ((oversized clothes)), brown shoes,lime-green very long sleeves,arms behind back ",
"negative_prompt": "bad-hands-5, (worst quality:2), (low quality:2),EasyNegative,lowres, ((1girl,fur trim,bangs,((hair)),((limes,sash)),underwear,necklace,choker,grass,motor vehicle,car,buttons,holding:1.2,monochrome,bad eyes,bad hands,underwear)), ((grayscale)",
"scheduler": "dpmpp_sde_ancestral",
"num_inference_steps": 25,
"guidance_scale": 9,
"samples": 1,
"seed": 5735283,
"img_width": 512,
"img_height": 768,
"base64": False
}
headers = {'x-api-key': api_key}
response = requests.post(url, json=data, headers=headers)
print(response.content) # The response is the generated image
Attributes
Prompt to render
Prompts to exclude, eg. 'bad anatomy, bad hands, missing fingers'
Type of scheduler.
Allowed values:
Number of denoising steps.
min : 20,
max : 100
Scale for classifier-free guidance
min : 0.1,
max : 25
Number of samples to generate.
min : 1,
max : 4
Seed for image generation.
Width of the image.
Allowed values:
Height of the Image
Allowed values:
Base64 encoding of the output image.
To keep track of your credit usage, you can inspect the response headers of each API call. The x-remaining-credits property will indicate the number of remaining credits in your account. Ensure you monitor this value to avoid any disruptions in your API usage.
Disney
Stable Diffusion Disney AI model is a latent diffusion model that can be used to generate images from text prompts. It is a powerful tool for AI developers who want to experiment with creative text-to-image generation, especially for generating images in the style of Disney movies.
The model is trained on a massive dataset of images and text from Disney movies, and it is specifically designed to generate images that have the same kind of vibrant colors, expressive characters, and whimsical settings that are found in Disney movies. To use the model, you first need to provide a text prompt. The text prompt can be anything you want, such as a description of an image, a concept, or even just a few words.
Here are some tips for using Stable Diffusion Disney:
-
Use clear and concise text prompts. The more specific your text prompt is, the more likely the model is to generate an image that matches your expectations.
-
Experiment with different styles. Stable Diffusion Disney AI model can generate images in a variety of styles. Try different text prompts to see how the model generates different styles of images.
-
Adjust the number of diffusion steps. The number of diffusion steps controls the level of detail in the image. More diffusion steps will result in a more detailed image, but it will also take longer to generate the image.
Applications/Use Cases
-
Generating concept art based Disney movies.
-
Creating fan art based on Disney movies.
-
Designing merchandise based on Disney movies.
Other Popular Models
sdxl-controlnet
SDXL ControlNet gives unprecedented control over text-to-image generation. SDXL ControlNet models Introduces the concept of conditioning inputs, which provide additional information to guide the image generation process
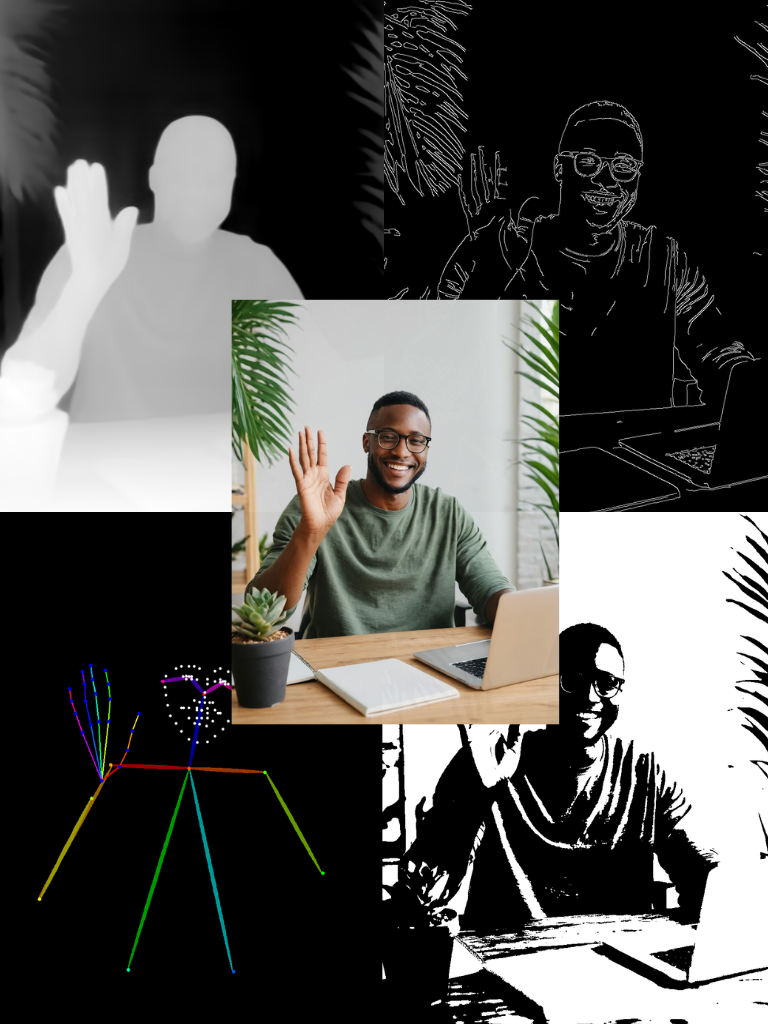
sdxl-inpaint
This model is capable of generating photo-realistic images given any text input, with the extra capability of inpainting the pictures by using a mask
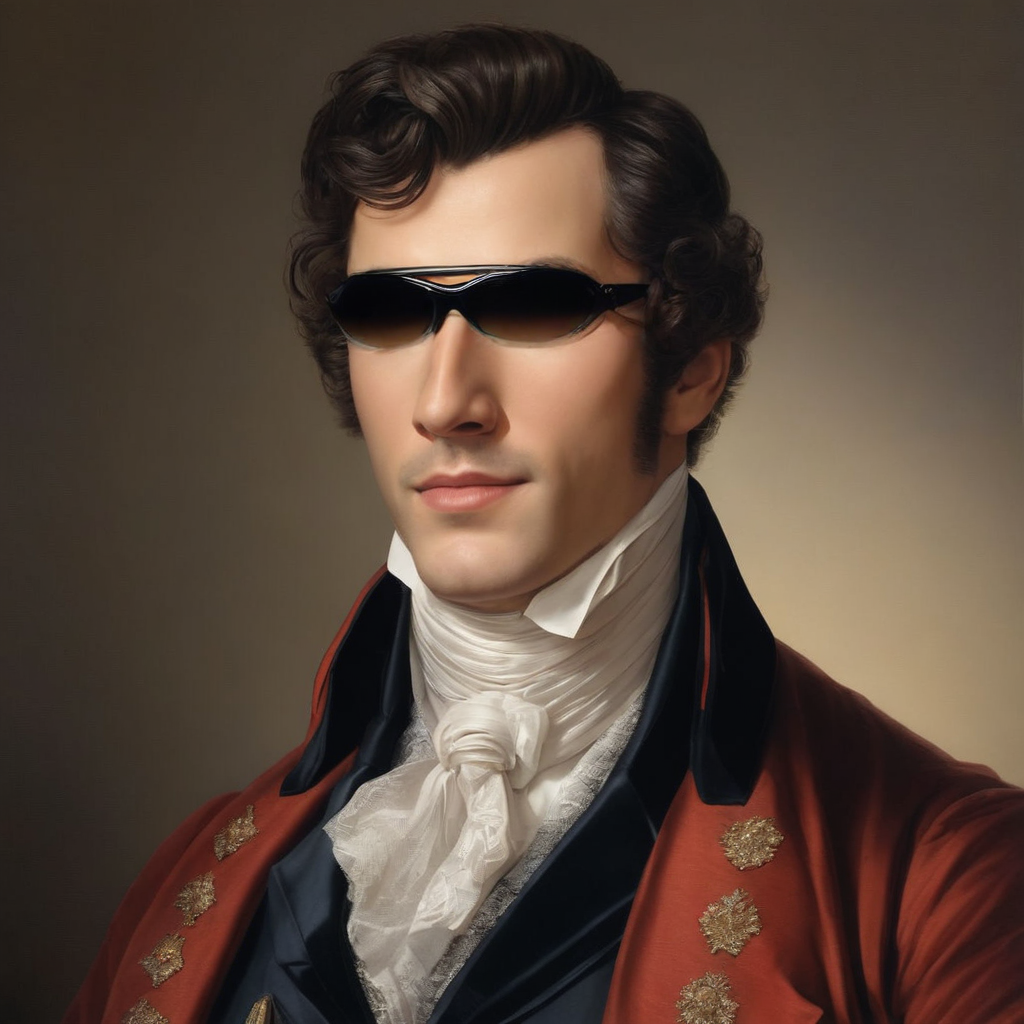
sdxl1.0-txt2img
The SDXL model is the official upgrade to the v1.5 model. The model is released as open-source software
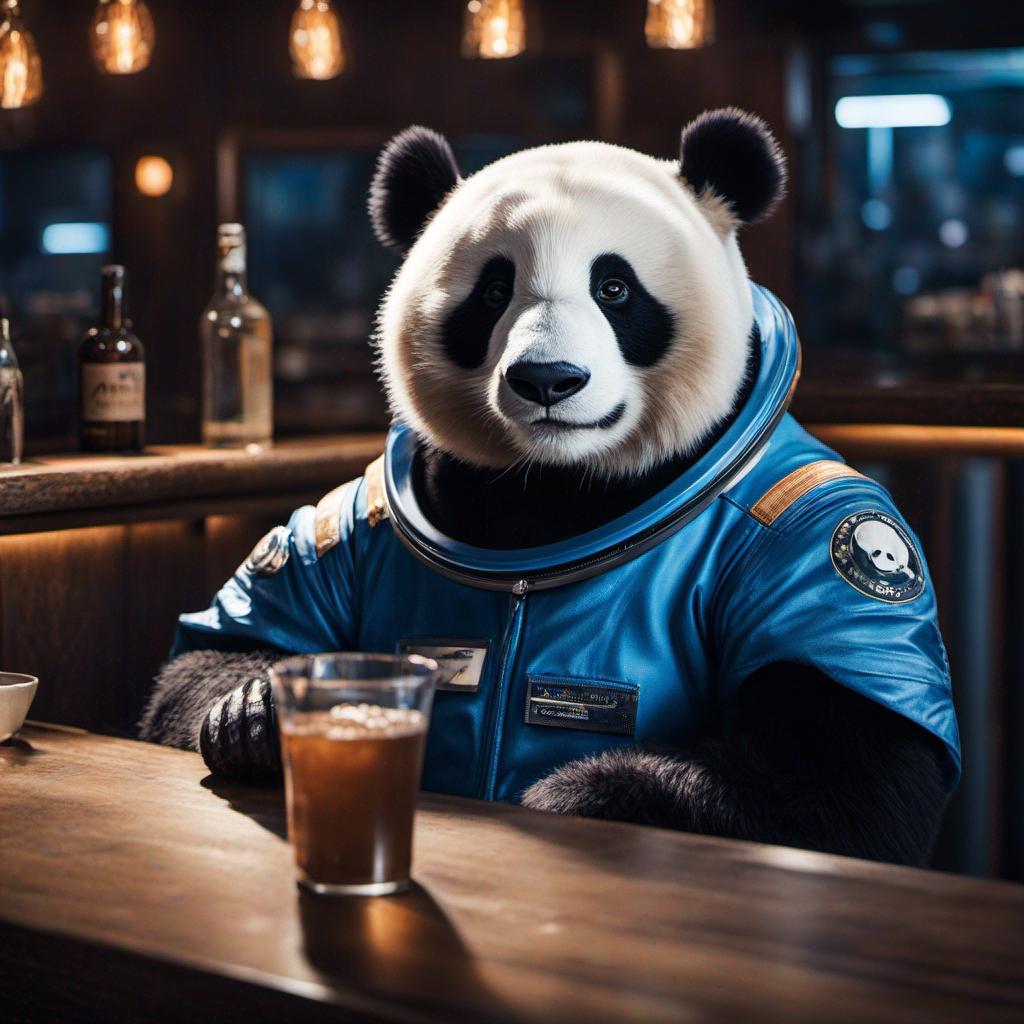
sd2.1-faceswapper
Take a picture/gif and replace the face in it with a face of your choice. You only need one image of the desired face. No dataset, no training
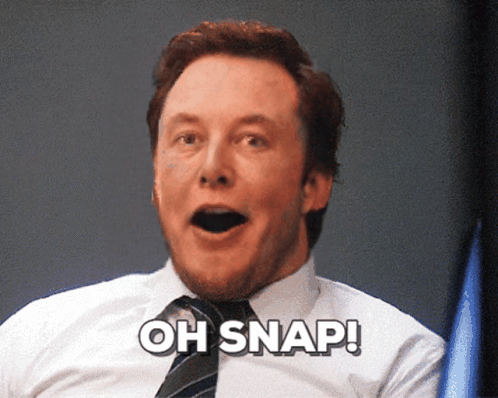