API
If you're looking for an API, you can choose from your desired programming language.
1
2
3
4
5
6
7
8
9
10
11
12
13
14
15
16
17
18
19
20
21
22
23
24
25
26
27
28
29
30
31
32
33
34
35
36
37
38
39
40
import requests
import base64
# Use this function to convert an image file from the filesystem to base64
def image_file_to_base64(image_path):
with open(image_path, 'rb') as f:
image_data = f.read()
return base64.b64encode(image_data).decode('utf-8')
# Use this function to fetch an image from a URL and convert it to base64
def image_url_to_base64(image_url):
response = requests.get(image_url)
image_data = response.content
return base64.b64encode(image_data).decode('utf-8')
# Use this function to convert a list of image URLs to base64
def image_urls_to_base64(image_urls):
return [image_url_to_base64(url) for url in image_urls]
api_key = "YOUR_API_KEY"
url = "https://api.segmind.com/v1/sd1.5-dvrach"
# Request payload
data = {
"prompt": "dvArchModern, 85mm, f1.8, portrait, photo realistic, hyperrealistic, orante, super detailed, intricate, dramatic, sun lighting, shadows, high dynamic range, modern interior, beach house, mexico",
"negative_prompt": "signature, soft, single floor,unclear, watermark, blurry, drawing, sketch, poor quality, ugly, text, type, word, logo, pixelated, low resolution, saturated, high contrast, oversharpened",
"scheduler": "euler_a",
"num_inference_steps": 20,
"guidance_scale": 7,
"samples": 1,
"seed": 5499784543,
"img_width": 512,
"img_height": 768,
"base64": False
}
headers = {'x-api-key': api_key}
response = requests.post(url, json=data, headers=headers)
print(response.content) # The response is the generated image
Attributes
Prompt to render
Prompts to exclude, eg. 'bad anatomy, bad hands, missing fingers'
Type of scheduler.
Allowed values:
Number of denoising steps.
min : 20,
max : 100
Scale for classifier-free guidance
min : 0.1,
max : 25
Number of samples to generate.
min : 1,
max : 4
Seed for image generation.
Width of the image.
Allowed values:
Height of the Image
Allowed values:
Base64 encoding of the output image.
To keep track of your credit usage, you can inspect the response headers of each API call. The x-remaining-credits property will indicate the number of remaining credits in your account. Ensure you monitor this value to avoid any disruptions in your API usage.
DvArch
DvArch, a bespoke trained model tailored to breathe life into architectural visions. With its unique approach of utilizing three distinct trigger words - dvArchModern, dvArchGothic, and dvArchVictorian - dvArch offers users a specialized avenue to explore and manifest architectural styles spanning centuries.
At the core of DvArch lies a sophisticated mechanism designed to respond to specific trigger words. Each trigger word acts as a gateway to a distinct architectural style, ensuring that users can seamlessly transition between modern minimalism, the grandeur of Gothic, and the elegance of Victorian, all within a single model framework.
Advantages
-
Tailored Outputs: The model's response to individual trigger words ensures designs that resonate with the chosen architectural style.
-
Versatile Design Palette: From the sleek lines of modern architecture to the intricate details of Victorian designs, DvArch offers a broad spectrum of design possibilities.
-
User-Centric Approach: The trigger word mechanism provides an intuitive user experience, allowing for easy navigation between styles.
-
High Precision: DvArch's custom training ensures accurate and authentic architectural renderings aligned with the chosen style.
-
Innovative Integration: The model's unique approach sets it apart, making it a valuable tool for architects and designers seeking specialized outputs.
Use Cases
-
Architectural Visualization: Architects can harness DvArch to visualize structures in various styles, aiding client presentations and design iterations.
-
Educational Platforms: Students and enthusiasts can explore architectural styles in-depth, understanding nuances and design principles.
-
Virtual Tours: Real estate and travel platforms can use DvArch to create immersive virtual tours, showcasing properties in different architectural renditions.
-
Game Design: Game developers can integrate DvArch to craft diverse in-game structures, enhancing world-building and player immersion.
-
Media and Film Production: Film producers and directors can utilize the model for pre-visualization, setting the tone for scenes and backdrops.
Other Popular Models
sdxl-img2img
SDXL Img2Img is used for text-guided image-to-image translation. This model uses the weights from Stable Diffusion to generate new images from an input image using StableDiffusionImg2ImgPipeline from diffusers
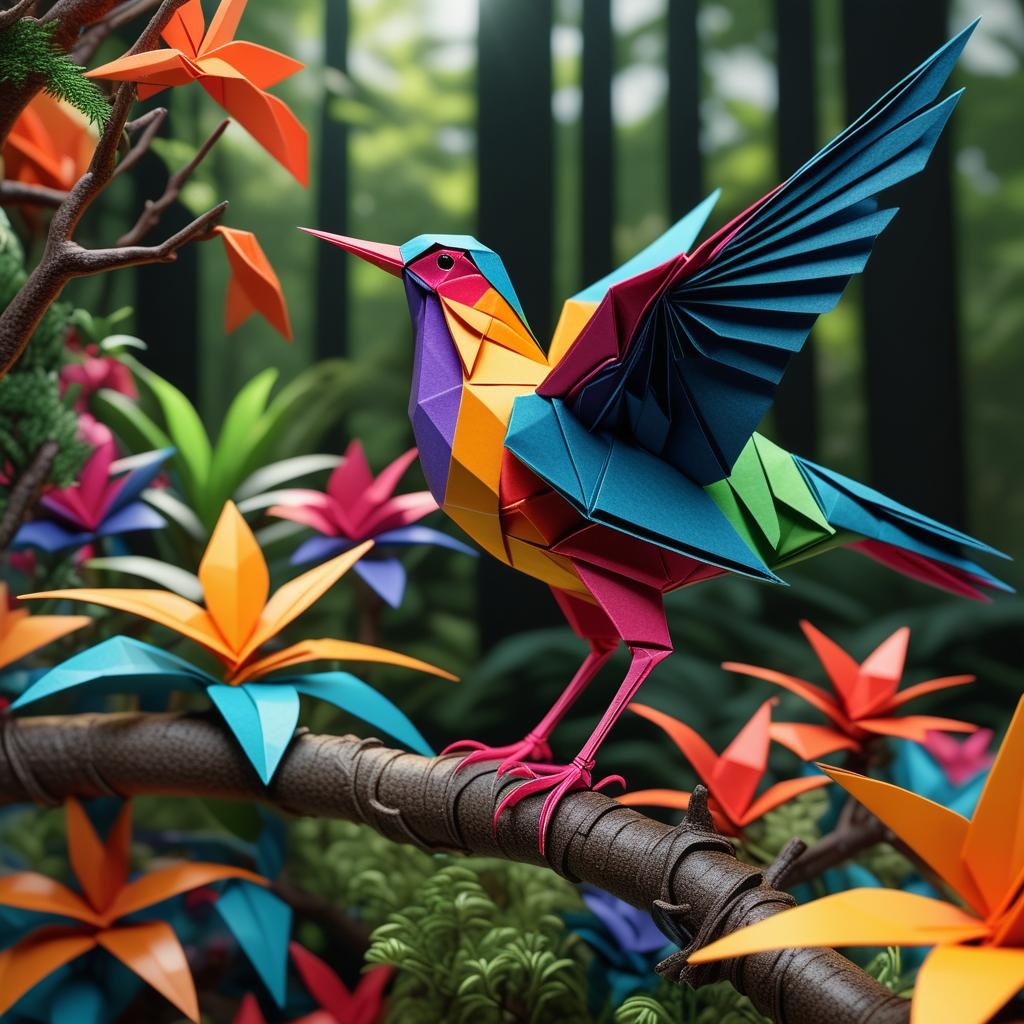
storydiffusion
Story Diffusion turns your written narratives into stunning image sequences.
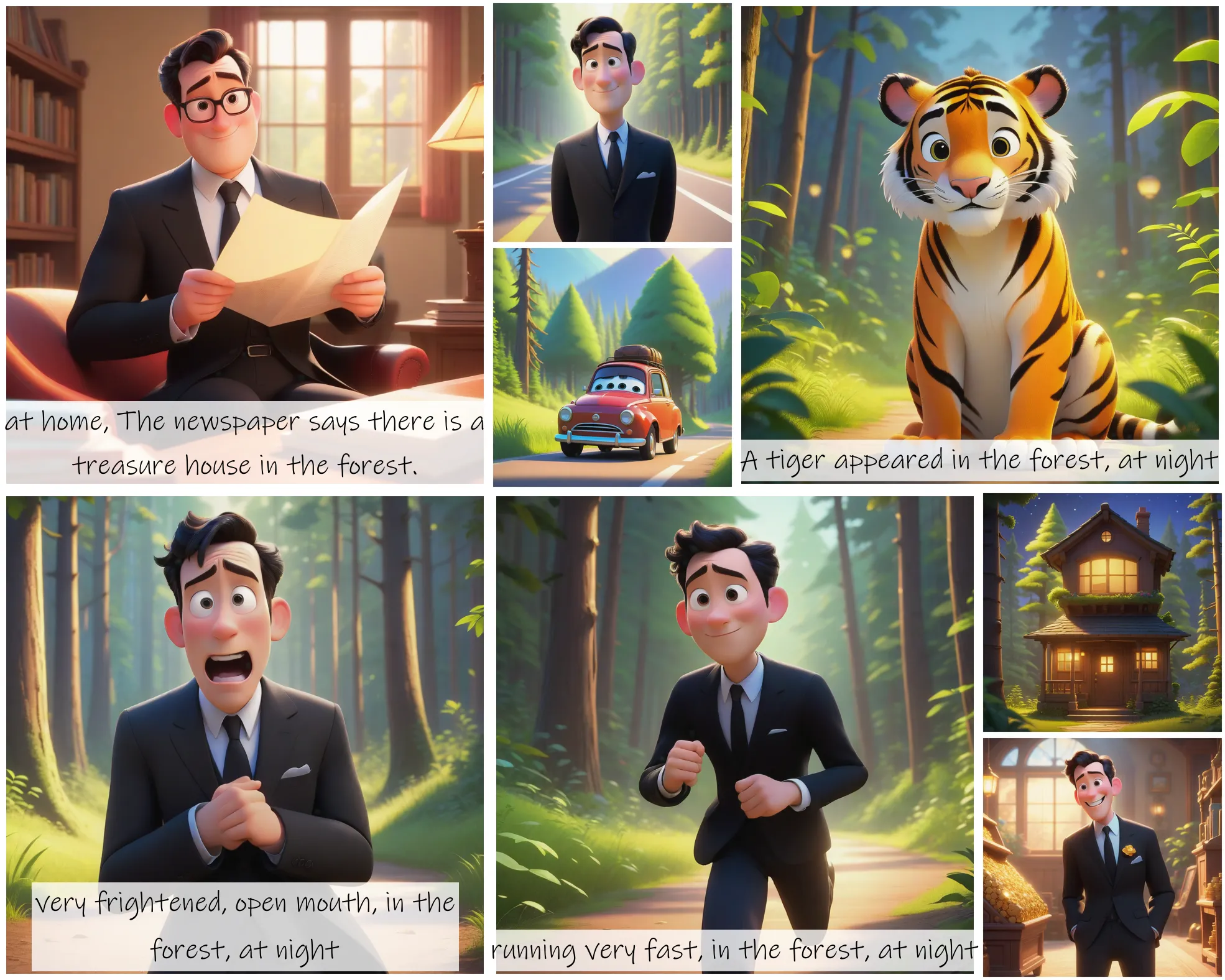
sadtalker
Audio-based Lip Synchronization for Talking Head Video
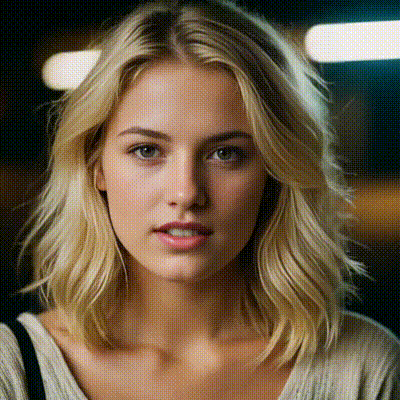
faceswap-v2
Take a picture/gif and replace the face in it with a face of your choice. You only need one image of the desired face. No dataset, no training
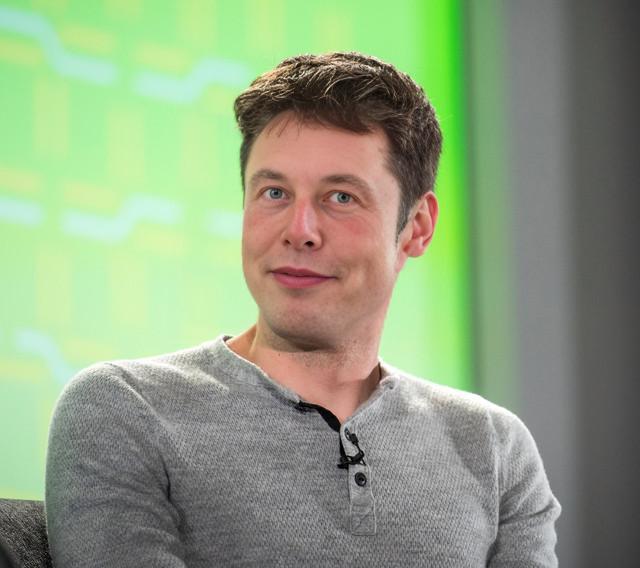