API
If you're looking for an API, you can choose from your desired programming language.
1
2
3
4
5
6
7
8
9
10
11
12
13
14
15
16
17
18
19
20
21
22
23
24
25
26
27
28
29
30
31
32
33
34
35
36
37
38
39
40
41
42
43
44
import requests
import base64
# Use this function to convert an image file from the filesystem to base64
def image_file_to_base64(image_path):
with open(image_path, 'rb') as f:
image_data = f.read()
return base64.b64encode(image_data).decode('utf-8')
# Use this function to fetch an image from a URL and convert it to base64
def image_url_to_base64(image_url):
response = requests.get(image_url)
image_data = response.content
return base64.b64encode(image_data).decode('utf-8')
api_key = "YOUR_API_KEY"
url = "https://api.segmind.com/v1/clarity-upscaler"
# Request payload
data = {
"seed": 1337,
"image": image_url_to_base64("https://segmind-sd-models.s3.amazonaws.com/display_images/clarity_upscale_input.png"), # Or use image_file_to_base64("IMAGE_PATH")
"prompt": "masterpiece, best quality, highres, <lora:more_details:0.5> <lora:SDXLrender_v2.0:1>",
"dynamic": 6,
"handfix": "disabled",
"sharpen": 0,
"sd_model": "juggernaut_reborn.safetensors [338b85bc4f]",
"scheduler": "DPM++ 3M SDE Karras",
"creativity": 0.35,
"downscaling": False,
"resemblance": 0.6,
"scale_factor": 1,
"tiling_width": 112,
"output_format": "png",
"tiling_height": 144,
"negative_prompt": "(worst quality, low quality, normal quality:2) JuggernautNegative-neg",
"num_inference_steps": 18,
"downscaling_resolution": 768
}
headers = {'x-api-key': api_key}
response = requests.post(url, json=data, headers=headers)
print(response.content) # The response is the generated image
Attributes
Random seed. Leave blank to randomize the seed
input image
Mask image to mark areas that should be preserved during upscaling
Prompt
HDR, try from 3 - 9
min : 1,
max : 50
An enumeration.
Allowed values:
Sharpen the image after upscaling. The higher the value, the more sharpening is applied. 0 for no sharpening
min : 0,
max : 10
An enumeration.
Allowed values:
An enumeration.
Allowed values:
Creativity, try from 0.3 - 0.9
min : 0,
max : 1
Link to a lora file you want to use in your upscaling. Multiple links possible, seperated by comma
Downscale the image before upscaling. Can improve quality and speed for images with high resolution but lower quality
Resemblance, try from 0.3 - 1.6
min : 0,
max : 3
Scale factor
An enumeration.
Allowed values:
An enumeration.
Allowed values:
An enumeration.
Allowed values:
Negative Prompt
Number of denoising steps
min : 1,
max : 100
Downscaling resolution
To keep track of your credit usage, you can inspect the response headers of each API call. The x-remaining-credits property will indicate the number of remaining credits in your account. Ensure you monitor this value to avoid any disruptions in your API usage.
Clarity Upscaler
Clarity Upscaler transforms blurry images into crisp, high-definition versions. This powerful tool analyzes each pixel within an image and uses machine learning to fill in missing information, effectively increasing the resolution. Beyond simple upscaling, Clarity Upscaler acts as an intelligent enhancer. It analyzes your photos and strategically adds details to improve image quality. This can include sharpening textures in landscapes or increasing clarity in portraits. The level of detail added is entirely customizable. Clarity Upscaler offers user control over the AI's influence, allowing you to tailor the final image to your specific needs. Restore faded memories, create high-quality social media content, or simply enhance your existing photos – Clarity Upscaler enables you to achieve professional-looking results with ease.
Other Popular Models
sdxl-img2img
SDXL Img2Img is used for text-guided image-to-image translation. This model uses the weights from Stable Diffusion to generate new images from an input image using StableDiffusionImg2ImgPipeline from diffusers
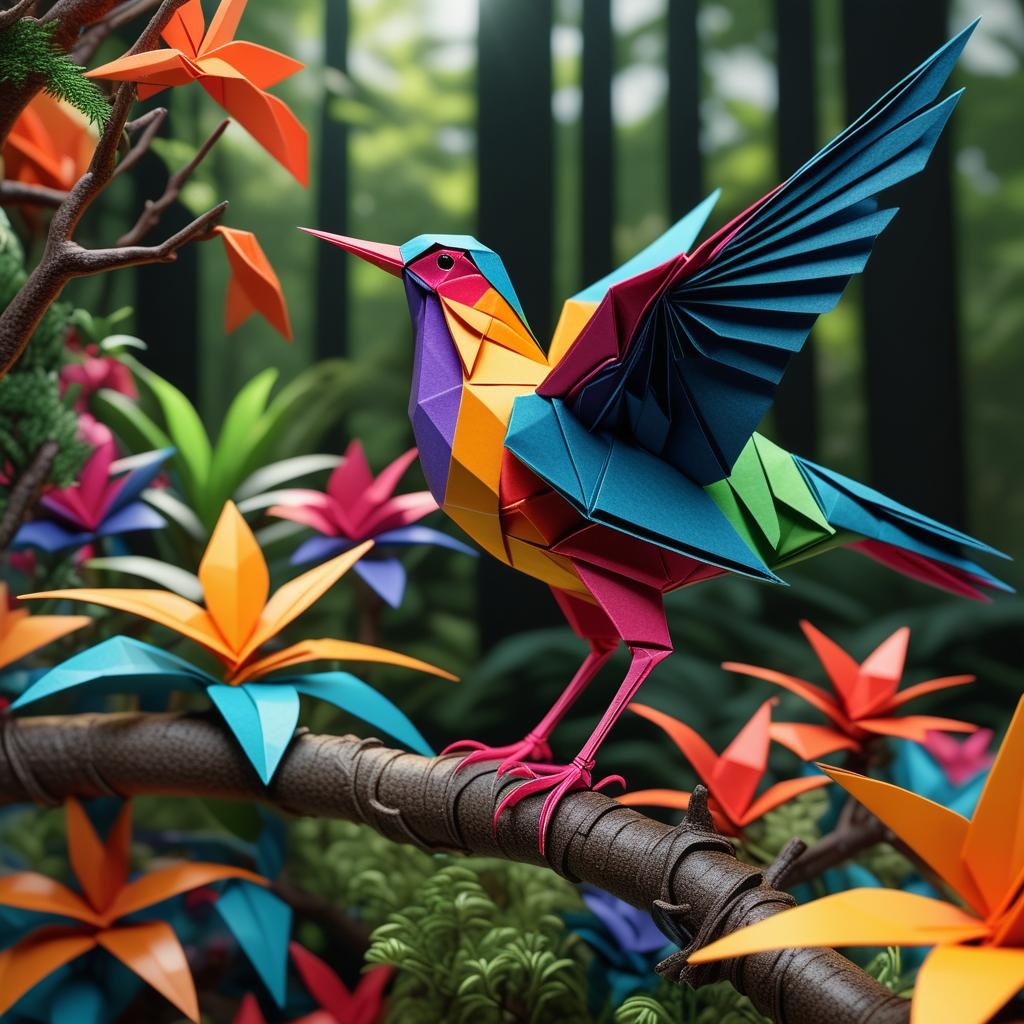
sdxl-controlnet
SDXL ControlNet gives unprecedented control over text-to-image generation. SDXL ControlNet models Introduces the concept of conditioning inputs, which provide additional information to guide the image generation process
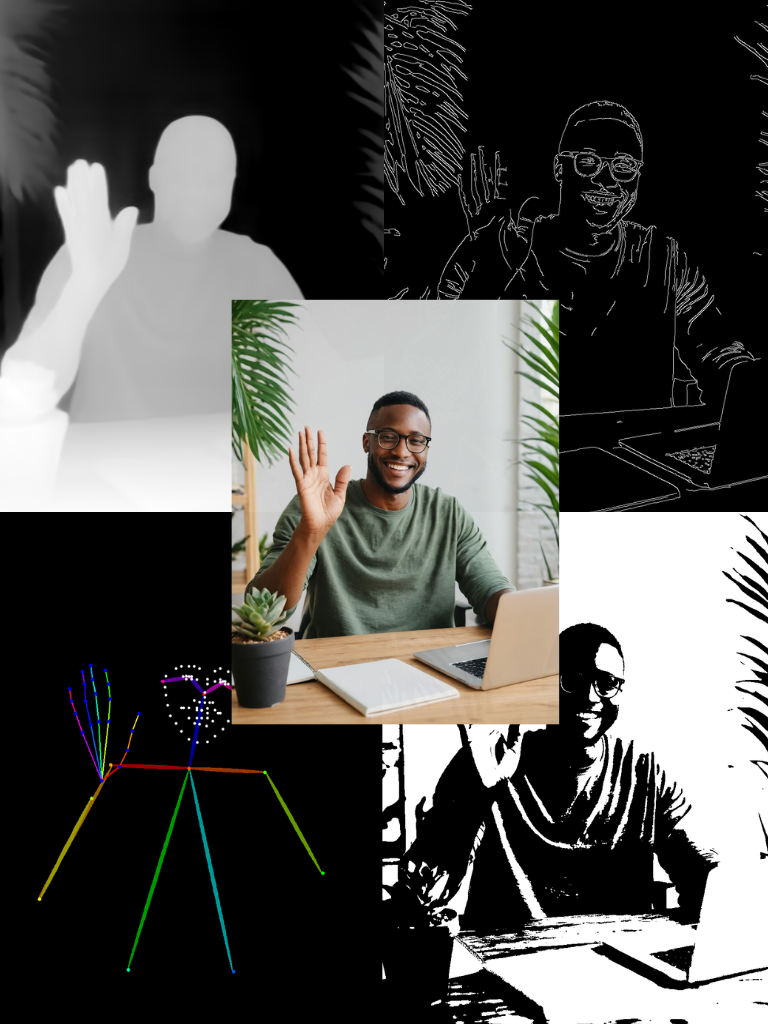
faceswap-v2
Take a picture/gif and replace the face in it with a face of your choice. You only need one image of the desired face. No dataset, no training
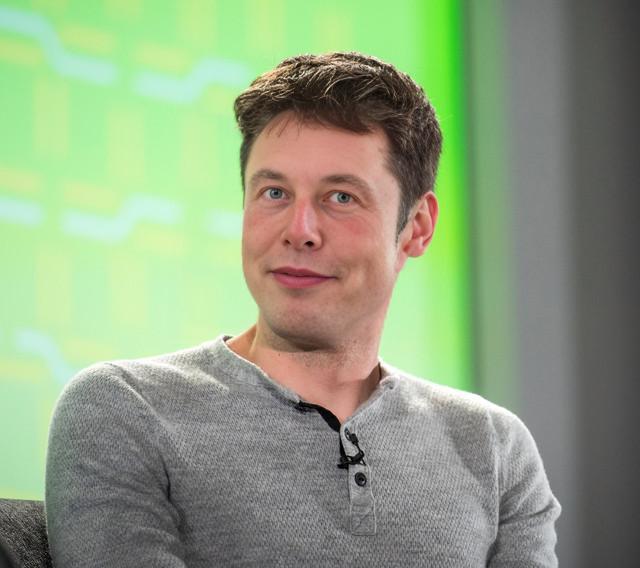
sdxl-inpaint
This model is capable of generating photo-realistic images given any text input, with the extra capability of inpainting the pictures by using a mask
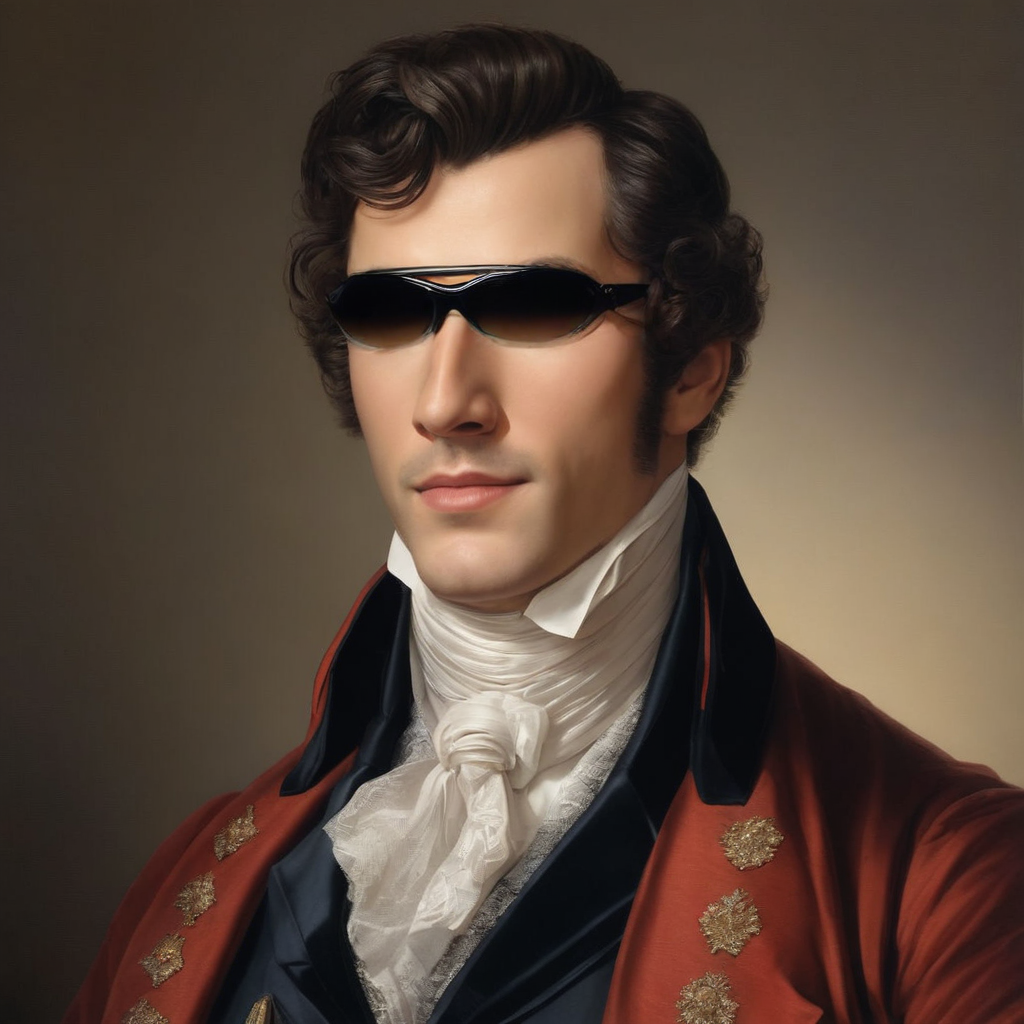