API
If you're looking for an API, you can choose from your desired programming language.
1
2
3
4
5
6
7
8
9
10
11
12
13
14
15
16
17
18
19
20
21
22
23
24
25
import requests
api_key = "YOUR_API_KEY"
url = "https://api.segmind.com/v1/hidream-l1-fast"
# Prepare data and files
data = {}
files = {}
data['seed'] = -1
data['prompt'] = "a cute panda holding a sign that says \"Keep Calm and Keep Building\""
data['model_type'] = "fast"
data['resolution'] = "1024 Ă— 1024 (Square)"
data['speed_mode'] = "Lightly Juiced 🍊 (more consistent)"
data['output_format'] = "webp"
data['output_quality'] = 100
headers = {'x-api-key': api_key}
# If no files, send as JSON
if files:
response = requests.post(url, data=data, files=files, headers=headers)
else:
response = requests.post(url, json=data, headers=headers)
print(response.content) # The response is the generated image
Attributes
Seed (-1 for random)
Prompt
An enumeration.
Allowed values:
Image resolution
Allowed values:
Quality vs Speed
Allowed values:
Output format.
Allowed values:
Output image quality (for jpg and webp)
min : 1,
max : 100
To keep track of your credit usage, you can inspect the response headers of each API call. The x-remaining-credits property will indicate the number of remaining credits in your account. Ensure you monitor this value to avoid any disruptions in your API usage.
Overview: HiDream-I1
HiDream-I1 is a state-of-the-art, open-source text-to-image model built for exceptional image generation quality, accurate prompt adherence, and broad commercial usability. It's designed for creators, developers, and researchers looking for high performance without licensing constraints.
Key Features
| Feature | Description | |-------------------------------|-------------| | Superior Image Quality | Consistently produces high-fidelity images across styles—photorealistic, cartoon, concept art, and more. Scores highly on the HPS v2.1 benchmark, which aligns with human aesthetic preferences. Great at rendering text within images. | | Best-in-Class Prompt Following | Achieves top-tier scores on GenEval and DPG benchmarks. Outperforms all other open-source models in prompt accuracy, ensuring precise visual outputs from user instructions. | | Open Source (MIT License) | Freely available for personal, academic, and commercial use. Ideal for developers and startups seeking to integrate a powerful model without licensing headaches. | | Commercial-Ready | Outputs can be used for business applications like product mockups, ads, UI/UX design, and content creation, without additional licensing requirements. | | Multiple Versions Available | Choose from: • Full – highest quality • Dev – quality-performance balance • Fast – optimized for real-time use |
Technical Highlights
| Component | Details | |------------------|---------| | Architecture | Based on Mixture of Experts (MoE) using a Diffusion Transformer (DiT) backbone for modular and efficient processing. | | Text Encoders | Integrates multiple encoders for richer semantic understanding: • OpenCLIP • OpenAI CLIP • T5-XXL • Llama-3.1-8B-Instruct | | Routing | Uses dynamic routing to selectively activate expert pathways based on the input prompt, boosting both quality and efficiency. |
Ideal Use Cases
- Concept art and storyboarding
- Product photography and eCommerce mockups
- Graphic design and editorial images
- Game asset creation
- UI/UX prototyping with text-in-image requirements
- Research and experimentation in generative AI
Other Popular Models
sdxl-img2img
SDXL Img2Img is used for text-guided image-to-image translation. This model uses the weights from Stable Diffusion to generate new images from an input image using StableDiffusionImg2ImgPipeline from diffusers
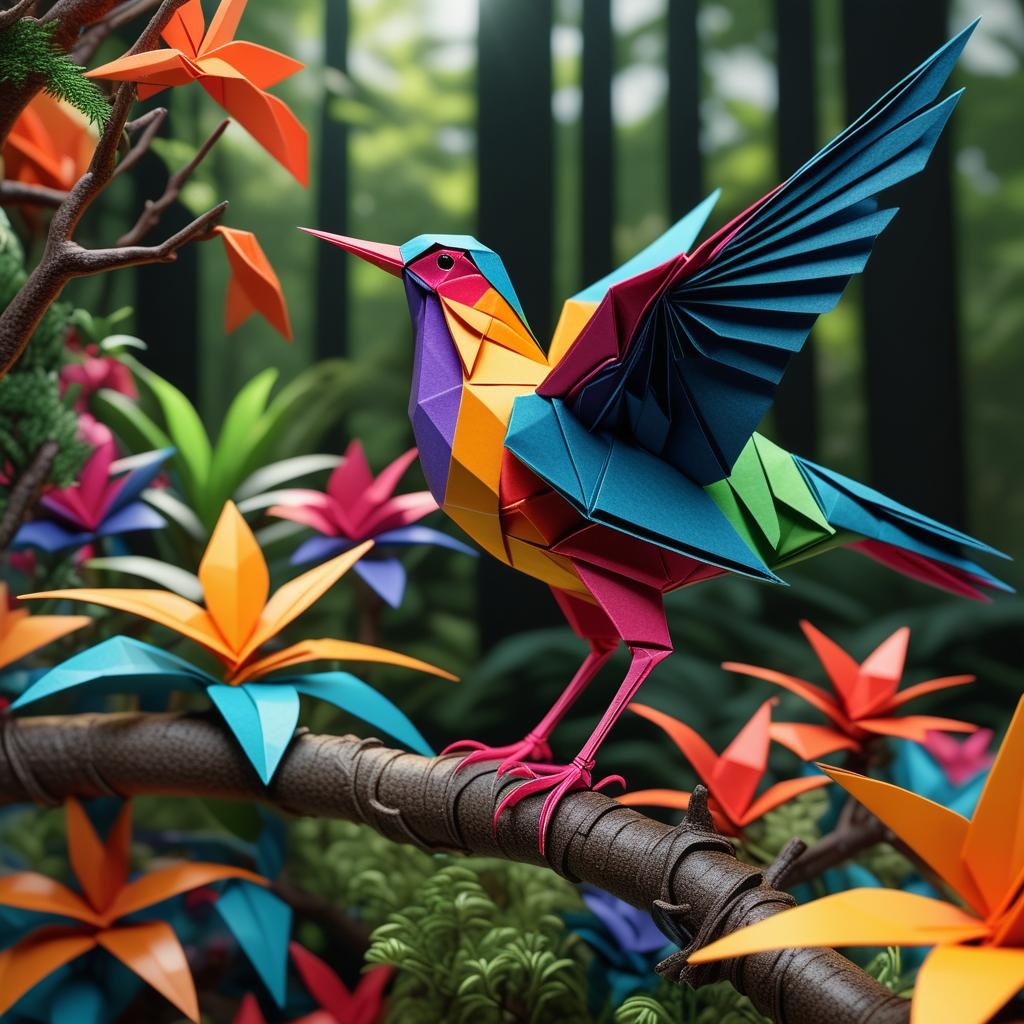
illusion-diffusion-hq
Monster Labs QrCode ControlNet on top of SD Realistic Vision v5.1
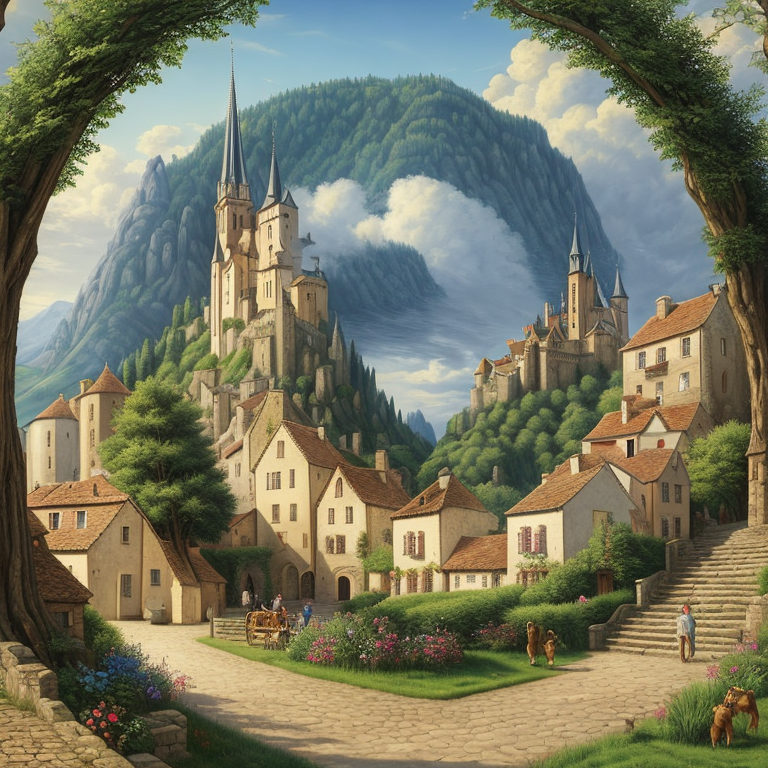
faceswap-v2
Take a picture/gif and replace the face in it with a face of your choice. You only need one image of the desired face. No dataset, no training
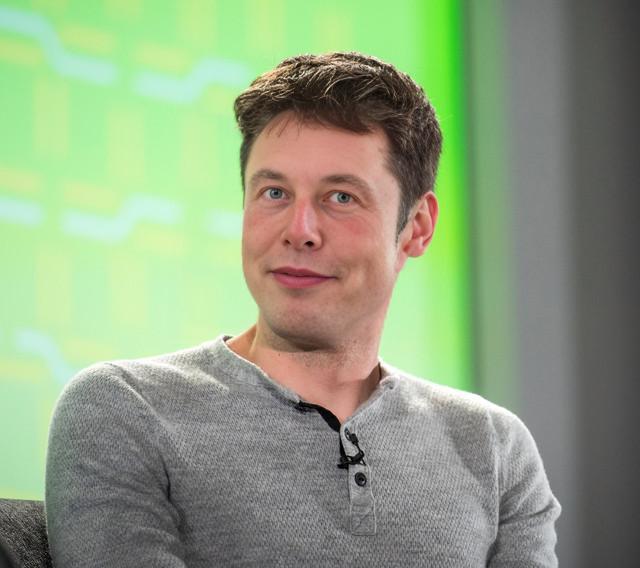
sd2.1-faceswapper
Take a picture/gif and replace the face in it with a face of your choice. You only need one image of the desired face. No dataset, no training
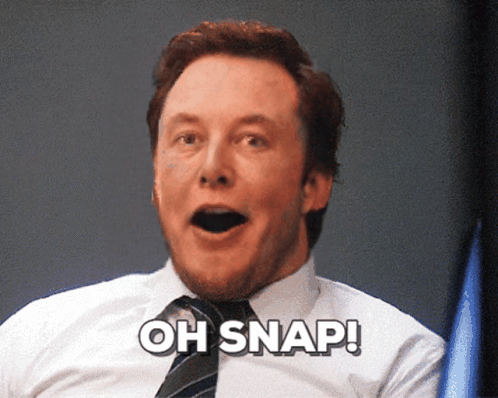