API
If you're looking for an API, you can choose from your desired programming language.
1
2
3
4
5
6
7
8
9
10
11
12
13
14
15
16
17
18
19
20
21
22
23
24
25
26
27
28
29
30
31
32
33
34
35
36
37
38
import requests
import base64
# Use this function to convert an image file from the filesystem to base64
def image_file_to_base64(image_path):
with open(image_path, 'rb') as f:
image_data = f.read()
return base64.b64encode(image_data).decode('utf-8')
# Use this function to fetch an image from a URL and convert it to base64
def image_url_to_base64(image_url):
response = requests.get(image_url)
image_data = response.content
return base64.b64encode(image_data).decode('utf-8')
api_key = "YOUR_API_KEY"
url = "https://api.segmind.com/v1/instantid"
# Request payload
data = {
"prompt": "photo of a man",
"face_image": image_url_to_base64("https://segmind-sd-models.s3.amazonaws.com/outputs/instantid_input.jpg"), # Or use image_file_to_base64("IMAGE_PATH")
"negative_prompt": "lowquality, badquality, sketches",
"style": "Vibrant Color",
"samples": 1,
"num_inference_steps": 10,
"guidance_scale": 5,
"seed": 354849415,
"identity_strength": 0.8,
"adapter_strength": 0.8,
"enhance_face_region": True,
"base64": False
}
headers = {'x-api-key': api_key}
response = requests.post(url, json=data, headers=headers)
print(response.content) # The response is the generated image
Attributes
Prompt to render
Face Image.
Pose Image.
Prompts to exclude, eg. 'bad anatomy, bad hands, missing fingers'
Style to apply
Number of samples to generate.
min : 1,
max : 4
Number of denoising steps.
min : 4,
max : 100
Scale for classifier-free guidance
min : 1,
max : 25
Seed for image generation.
min : -1,
max : 999999999999999
Identity Strength
min : 0,
max : 1
Adapter Strength
min : 0,
max : 1
Enhance Face region
Base64 encoding of the output image.
To keep track of your credit usage, you can inspect the response headers of each API call. The x-remaining-credits property will indicate the number of remaining credits in your account. Ensure you monitor this value to avoid any disruptions in your API usage.
InstantID
InstantID presents a revolutionary method for identity-preserving image generation, enabling the transformation of a single reference ID image into a diverse array of customized images. This innovative solution is underpinned by a novel face encoder and IdentityNet, which work in tandem to ensure the faithful preservation of the original identity across all generated images. The face encoder meticulously captures the intricate details of the reference image, thereby upholding high fidelity in the resulting images. Meanwhile, IdentityNet leverages strong semantic and weak spatial conditions, integrating facial and landmark images with textual prompts to steer the image generation process. This powerful diffusion model-based tool is ideal for users seeking to personalize images in various poses or styles while safeguarding the integrity of the original identity. InstantID's exceptional performance and efficiency make it highly advantageous for real-world applications where identity preservation is paramount.
This groundbreaking approach to image generation has significant implications for diverse fields, including personalized image synthesis, creative applications, and identity-sensitive scenarios. By enabling the generation of customized images from a single reference ID image, InstantID streamlines the image personalization process, eliminates the need for multiple reference images, and upholds the integrity of the original identity. Its versatility and effectiveness underscore its potential for various creative and practical applications.
Applications:
1. Photography and Portraiture: Create varied portraits from a single photograph, exploring different styles and poses.
2. Fashion and Design: Visualize how a person would look in different outfits or settings.
3. Entertainment and Media: Generate diverse character images for media production.
4. Personalization and Branding: Customize marketing materials with personalized images.
Other Popular Models
sdxl-img2img
SDXL Img2Img is used for text-guided image-to-image translation. This model uses the weights from Stable Diffusion to generate new images from an input image using StableDiffusionImg2ImgPipeline from diffusers
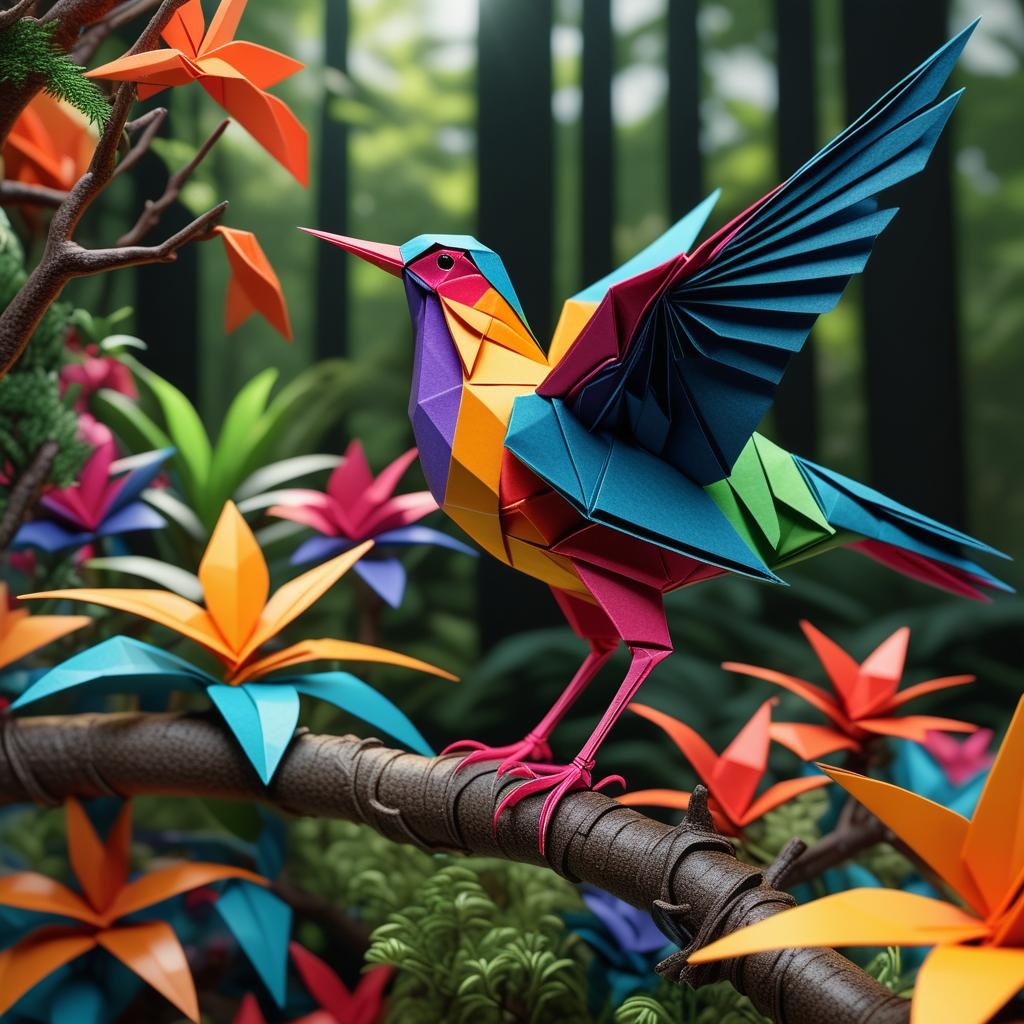
sdxl-controlnet
SDXL ControlNet gives unprecedented control over text-to-image generation. SDXL ControlNet models Introduces the concept of conditioning inputs, which provide additional information to guide the image generation process
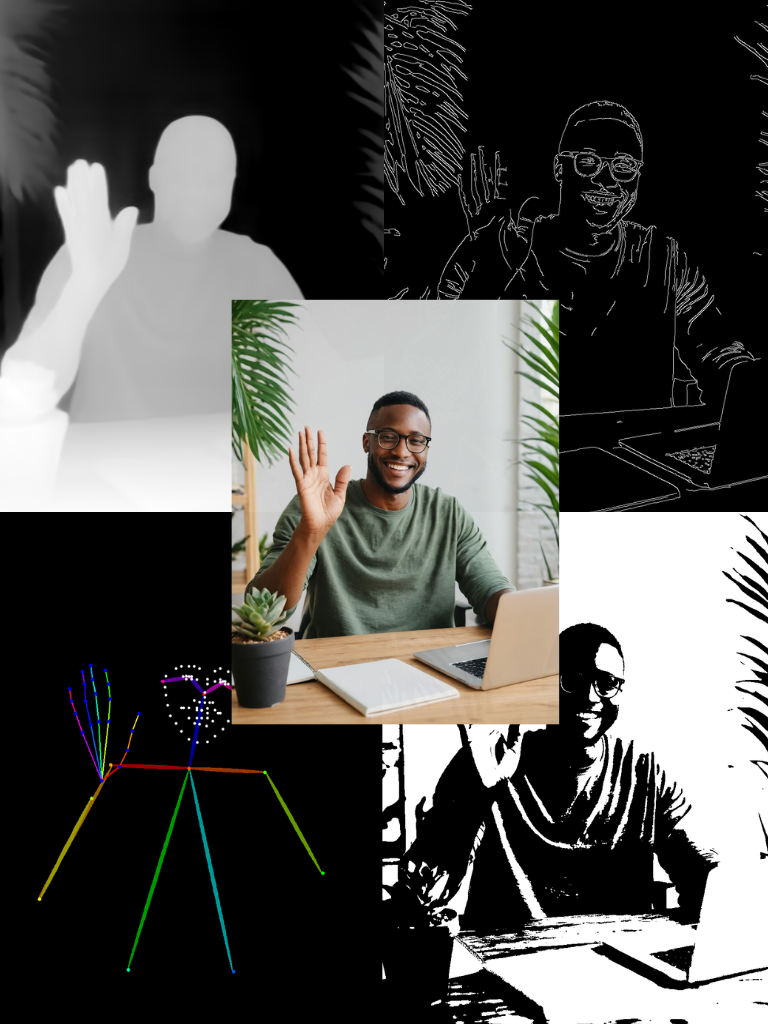
insta-depth
InstantID aims to generate customized images with various poses or styles from only a single reference ID image while ensuring high fidelity
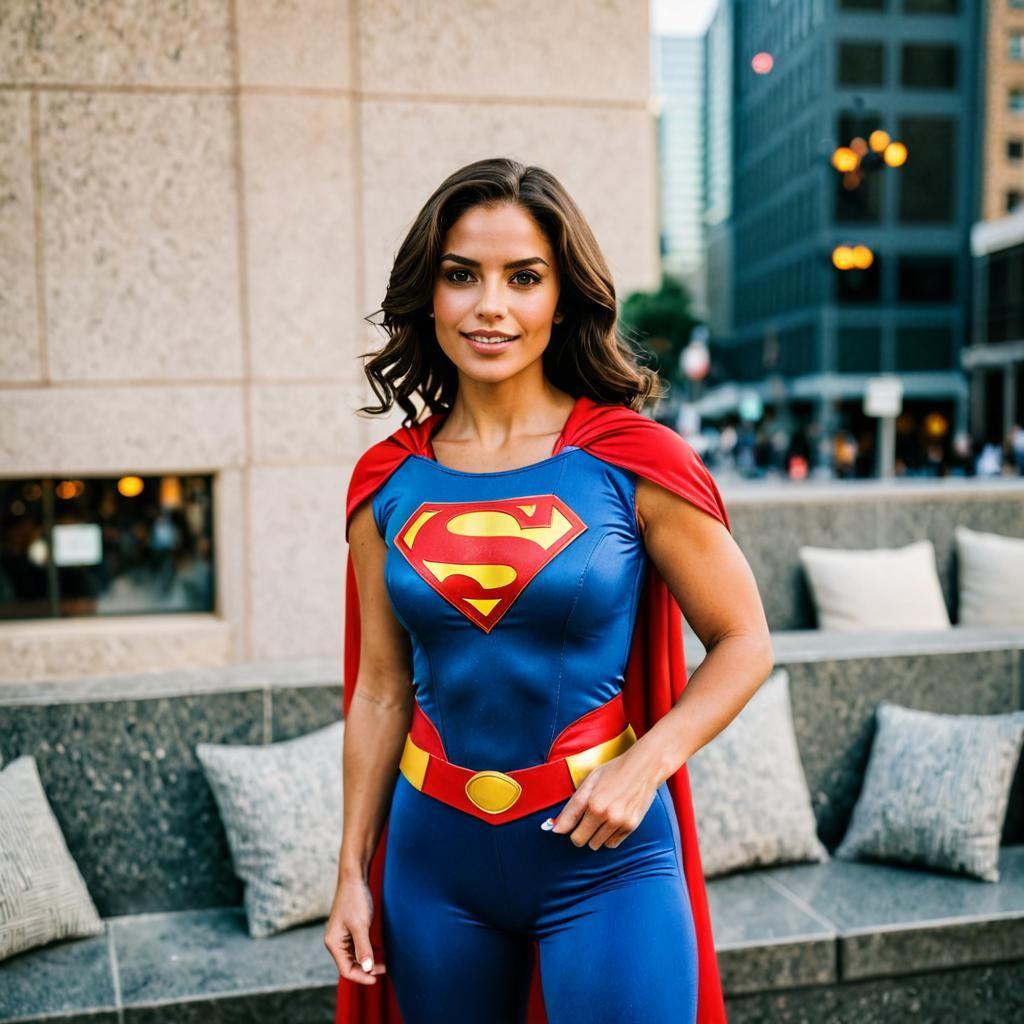
sd2.1-faceswapper
Take a picture/gif and replace the face in it with a face of your choice. You only need one image of the desired face. No dataset, no training
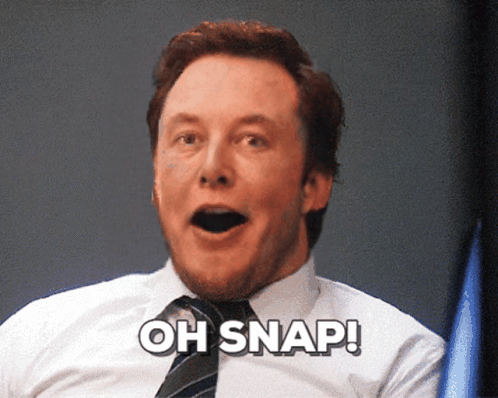