API
If you're looking for an API, you can choose from your desired programming language.
1
2
3
4
5
6
7
8
9
10
11
12
13
14
15
16
17
18
19
20
21
22
23
24
25
26
27
28
29
30
31
32
33
34
35
36
import requests
import base64
# Use this function to convert an image file from the filesystem to base64
def image_file_to_base64(image_path):
with open(image_path, 'rb') as f:
image_data = f.read()
return base64.b64encode(image_data).decode('utf-8')
# Use this function to fetch an image from a URL and convert it to base64
def image_url_to_base64(image_url):
response = requests.get(image_url)
image_data = response.content
return base64.b64encode(image_data).decode('utf-8')
api_key = "YOUR_API_KEY"
url = "https://api.segmind.com/v1/potraitsd1.5-txt2img"
# Request payload
data = {
"prompt": "RAW closeup portrait photo of a 25-year-old american, long brunette hair, pale skin, deep smile.",
"negative_prompt": "(deformed iris, deformed pupils, semi-realistic, cgi, render, sketch, cartoon, drawing, anime), text, cropped, out of frame, worst quality, low quality, ugly, tiling, poorly drawn hands, poorly drawn feet, poorly drawn face, out of frame, extra limbs, disfigured, deformed, body out of frame, blurry, bad anatomy, blurred, watermark, grainy, signature, cut off, draft, jpeg artifacts, ugly, duplicate, morbid, mutilated, extra fingers, mutated hands, poorly drawn hands, poorly drawn face, mutation, deformed, blurry, dehydrated, bad anatomy, bad proportions, extra limbs, cloned face, disfigured, gross proportions, malformed limbs, missing arms, missing legs, extra arms, extra legs, fused fingers, too many fingers, long neck, BadDream, UnrealisticDream",
"scheduler": "dpmpp_2m",
"num_inference_steps": 30,
"guidance_scale": 7.5,
"samples": 1,
"seed": 531327,
"img_width": 768,
"img_height": 768,
"base64": False
}
headers = {'x-api-key': api_key}
response = requests.post(url, json=data, headers=headers)
print(response.content) # The response is the generated image
Attributes
Prompt to render
Prompts to exclude, eg. 'bad anatomy, bad hands, missing fingers'
Type of scheduler.
Allowed values:
Number of denoising steps.
min : 20,
max : 100
Scale for classifier-free guidance
min : 0.1,
max : 25
Number of samples to generate.
min : 1,
max : 4
Seed for image generation.
Width of the image.
Allowed values:
Height of the Image
Allowed values:
Base64 encoding of the output image.
To keep track of your credit usage, you can inspect the response headers of each API call. The x-remaining-credits property will indicate the number of remaining credits in your account. Ensure you monitor this value to avoid any disruptions in your API usage.
Segmind Tiny-SD (Portrait): Bringing Lifelike Portraits to Life with AI
Welcome to Segmind Tiny-SD (Portrait), an exceptional innovation from Segmind that's revolutionizing the realm of generative AI models. This variant of the Tiny-SD model maintains the same compact size and high-speed performance, but it's specifically trained on human portrait prompts created using OpenAI's GPT4, and images generated by the Realistic Vision 4.0 model. This model is open-sourced and easily accessible on Huggingface, offering a fusion of superior quality and increased speed in portrait image generation.
The Segmind Tiny-SD (Portrait) leverages the power of Knowledge Distillation (KD) in its technical framework, mirroring the learning dynamics of a teacher-student relationship. A larger, pre-trained model (the teacher) supervises a compact model (the student) during training on a focused dataset of 7000 portrait images. This unique distillation methodology includes the principle of block-level output matching from the teacher model, ensuring the preservation of model quality even with significant size reduction. The distillation process hinges on a tri-component loss function that accounts for the traditional loss, the disparity between the teacher and student model's generated latients, and notably, the feature-level loss, a comparison of block outputs from both models.
The major benefit of the Segmind Tiny-SD (Portrait) model is its blend of rapidity, efficiency, and high-quality output. By offering up to 85% faster inferences, this model drastically trims down the time taken to produce results, while ensuring excellent image fidelity. Although it's a smaller model, its capabilities in generating high-quality portraits without any sacrifice in quality make it an optimal choice for tasks demanding fast and realistic portrait image synthesis.
Segmind Tiny-SD (Portrait) use cases
-
AI-Assisted Portrait Creation: Artists can generate high-quality and diverse human portraits quickly, enhancing their creative process.
-
Personalized Marketing: The model can be used for generating personalized portrait images for digital marketing campaigns, driving higher customer engagement.
-
Identity Verification Systems: It can be used for creating diverse datasets for training facial recognition or biometric verification systems.
-
Game Development: Game developers can leverage it for generating a diverse range of character portraits for more immersive gaming experiences.
-
Film and Animation: For filmmakers and animators, the model can rapidly generate a variety of character portraits, facilitating character design and storyboarding processes.
Segmind Tiny-SD (Portrait) License
Segmind Tiny-SD (Portrait) is licensed under CreativeML Open RAIL-M. This license encourages both the open and responsible use of the model. It is inspired by permissive open-source licenses in terms of granting IP rights while also adding use-based restrictions to prevent misuse of the technology, be it due to technical limitations or ethical considerations. While derivative versions of the model can be released under different licensing terms, they must always include the same use-based restrictions as the original license. Thus, the license strikes a balance between open and responsible AI development, promoting open-science in the field of AI while simultaneously addressing potential misuse.
Other Popular Models
storydiffusion
Story Diffusion turns your written narratives into stunning image sequences.
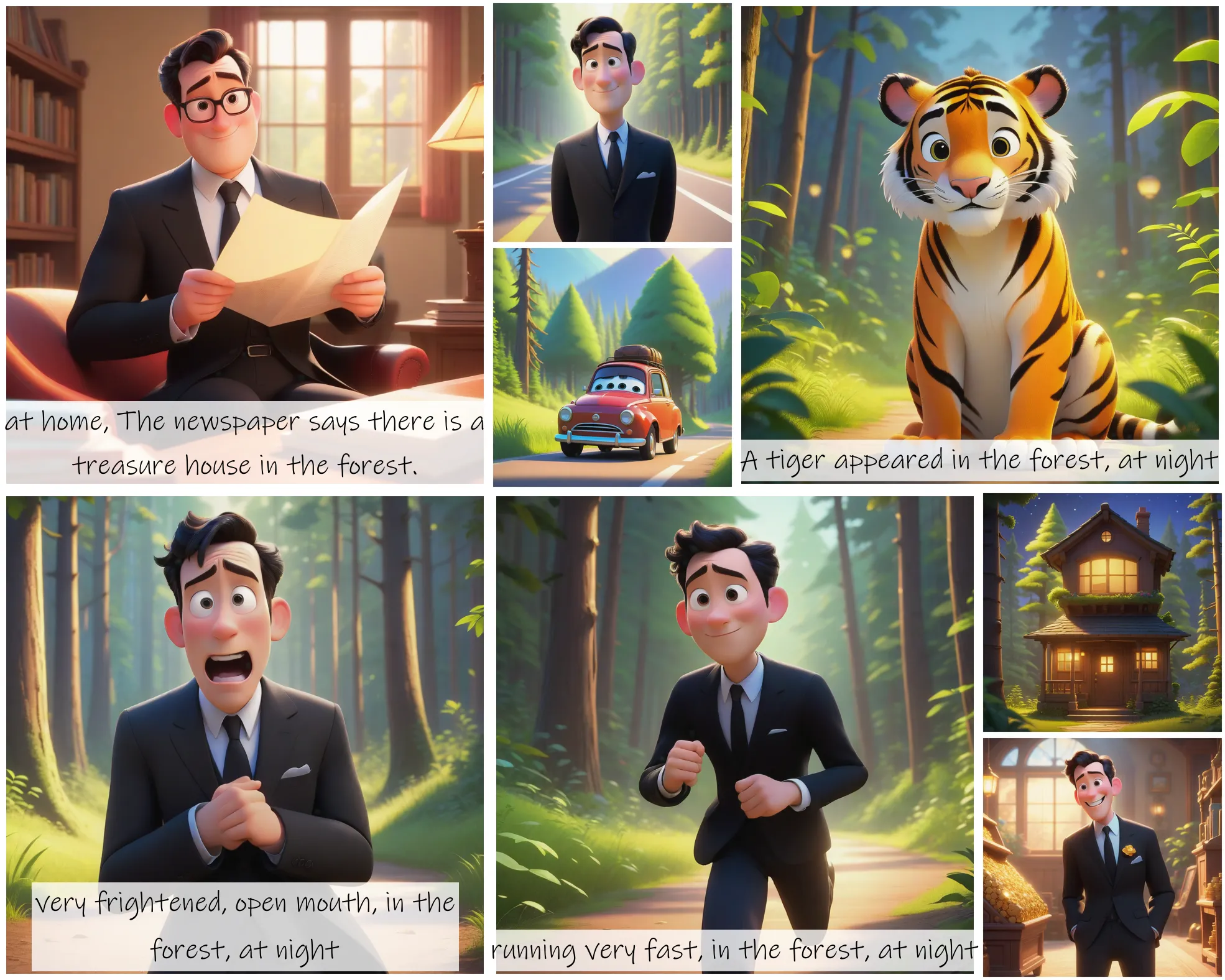
insta-depth
InstantID aims to generate customized images with various poses or styles from only a single reference ID image while ensuring high fidelity
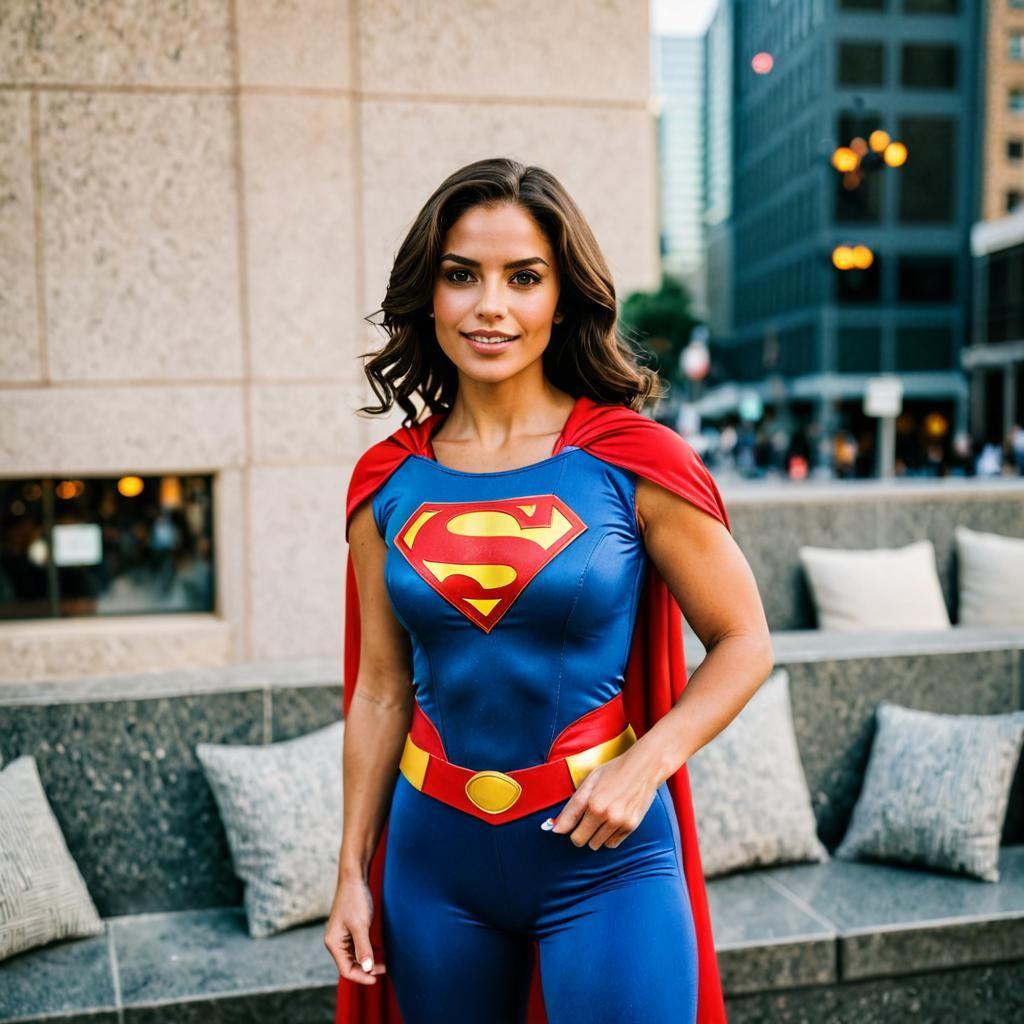
sdxl1.0-txt2img
The SDXL model is the official upgrade to the v1.5 model. The model is released as open-source software
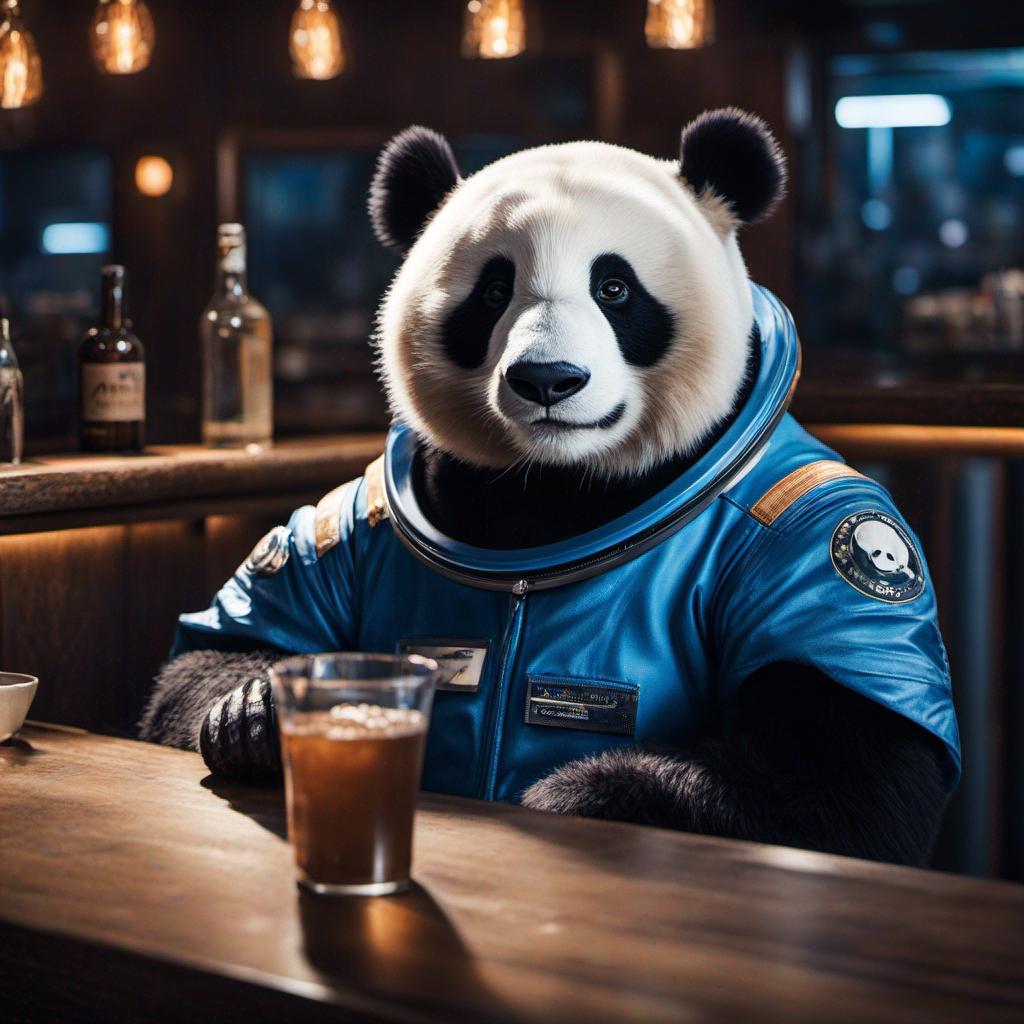
sd2.1-faceswapper
Take a picture/gif and replace the face in it with a face of your choice. You only need one image of the desired face. No dataset, no training
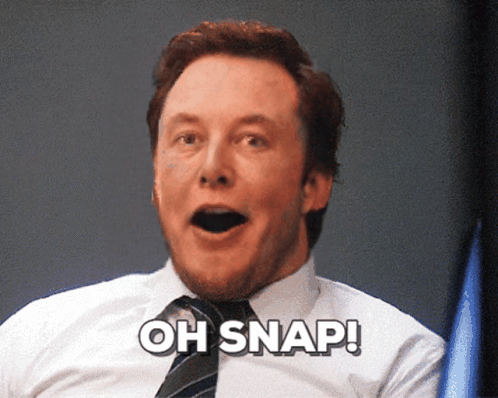