Qwen2-VL-72B-Instruct
Qwen2-VL-72B-Instruct is an advanced image-text-to-text model designed for a wide range of visual understanding and reasoning tasks. This model is a significant upgrade from the previous Qwen-VL, incorporating several key enhancement.
Key Features of Qwen2-VL-72B-Instruct
-
Superior Image Understanding: Qwen2-VL achieves state-of-the-art performance on various visual understanding benchmarks including MathVista, DocVQA, RealWorldQA, and MTVQA. It demonstrates strong capabilities in processing images with different resolutions and aspect ratios.
-
Agent Capabilities: Qwen2-VL can be integrated with devices like mobile phones and robots for automatic operation based on visual environment and text instructions, demonstrating complex reasoning and decision-making skills.
-
Multilingual Support: Beyond English and Chinese, the model supports understanding text within images in many languages, including most European languages, Japanese, Korean, Arabic, and Vietnamese.
-
Dynamic Resolution Handling: Qwen2-VL can handle arbitrary image resolutions, mapping them into a dynamic number of visual tokens for a more human-like visual processing experience.
-
Advanced Positional Embedding: The model uses Multimodal Rotary Position Embedding (M-ROPE) to capture 1D textual, 2D visual, and 3D video positional information, enhancing its multimodal processing capabilities
Technical Specifications
-
Model Architecture: The model employs a large-scale transformer architecture with 72 billion parameters.
-
Resolution Flexibility: The model is able to process a range of image resolutions, and its computational requirements can be adjusted by setting minimum and maximum pixel counts to optimize performance for specific hardware. Images can be resized to a specific width and height.
Limitations
-
The model has limitations in recognizing specific individuals or intellectual property.
-
It may struggle with complex, multi-step instructions.
-
Counting accuracy is not high in complex scenes.
-
Spatial reasoning skills, especially in 3D spaces, require further improvements.
Other Popular Models
sdxl-img2img
SDXL Img2Img is used for text-guided image-to-image translation. This model uses the weights from Stable Diffusion to generate new images from an input image using StableDiffusionImg2ImgPipeline from diffusers
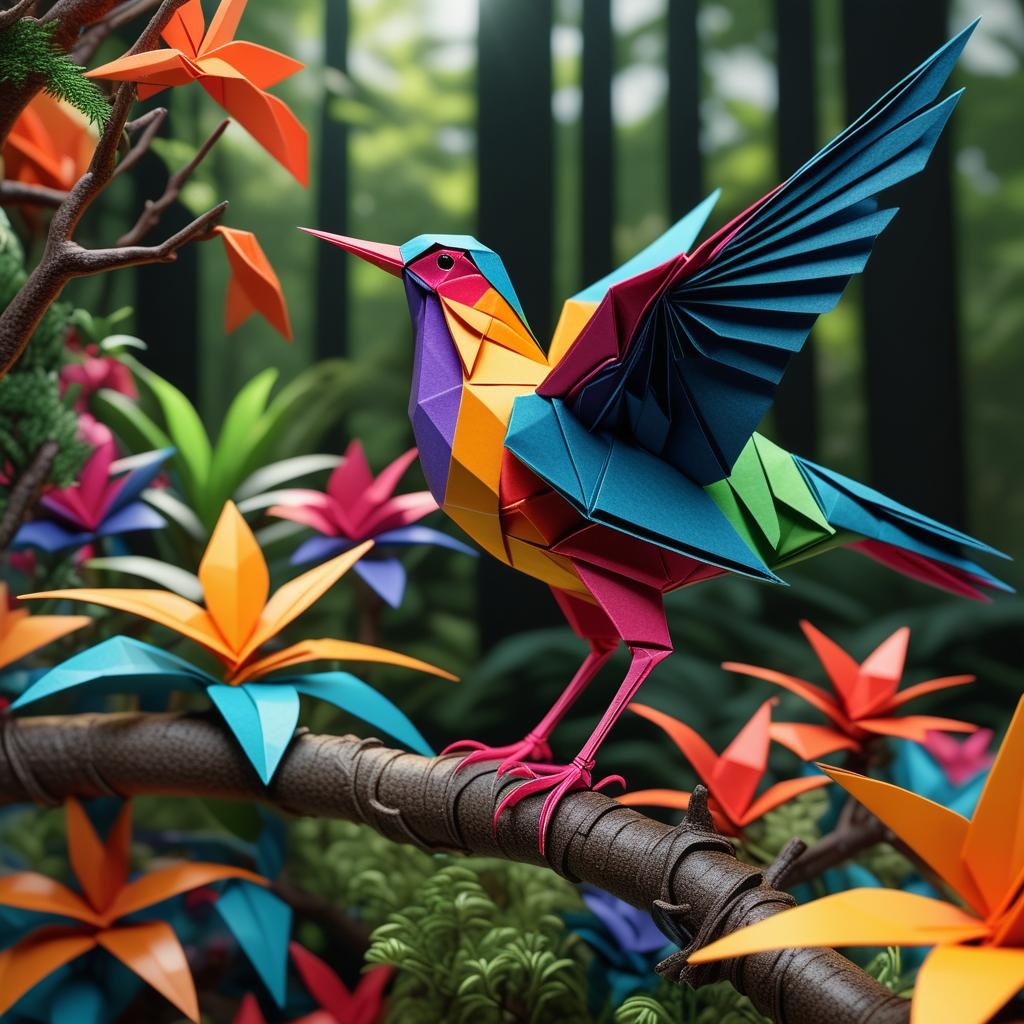
fooocus
Fooocus enables high-quality image generation effortlessly, combining the best of Stable Diffusion and Midjourney.
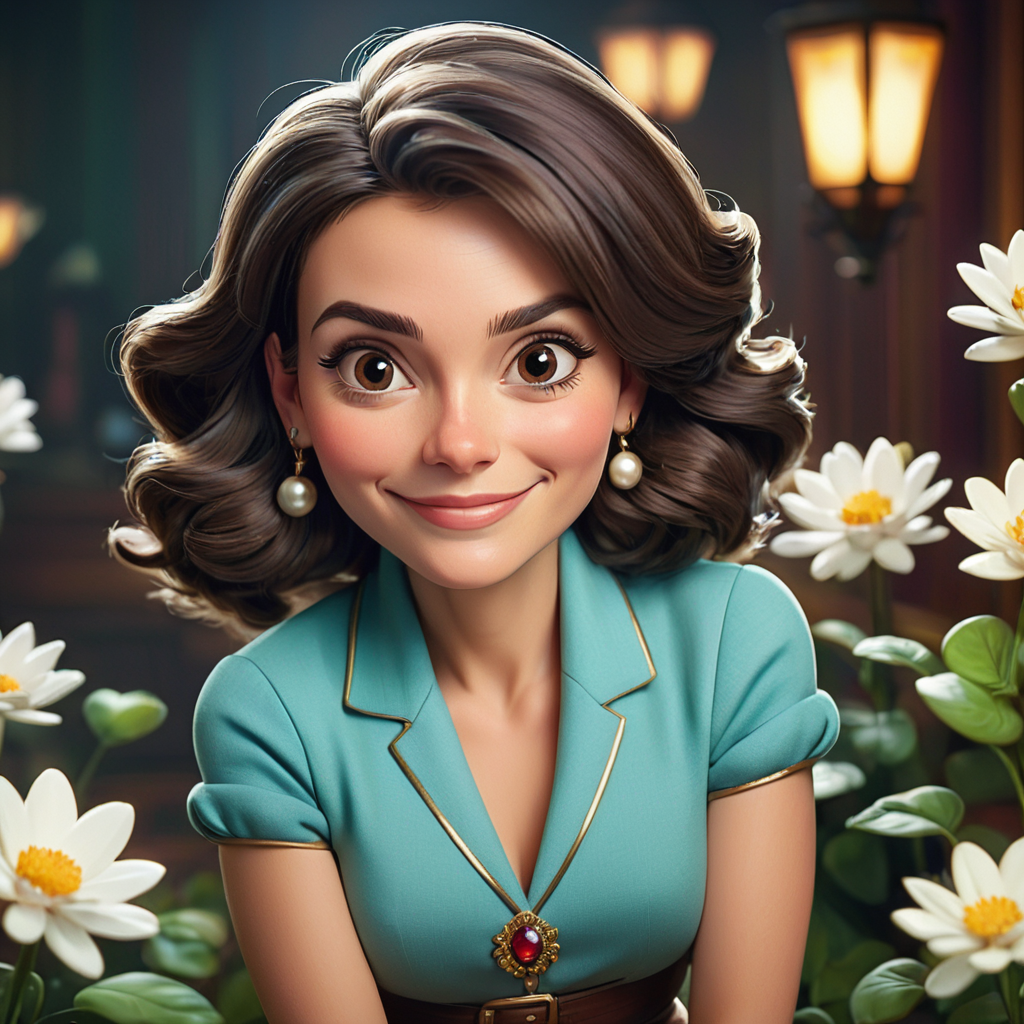
illusion-diffusion-hq
Monster Labs QrCode ControlNet on top of SD Realistic Vision v5.1
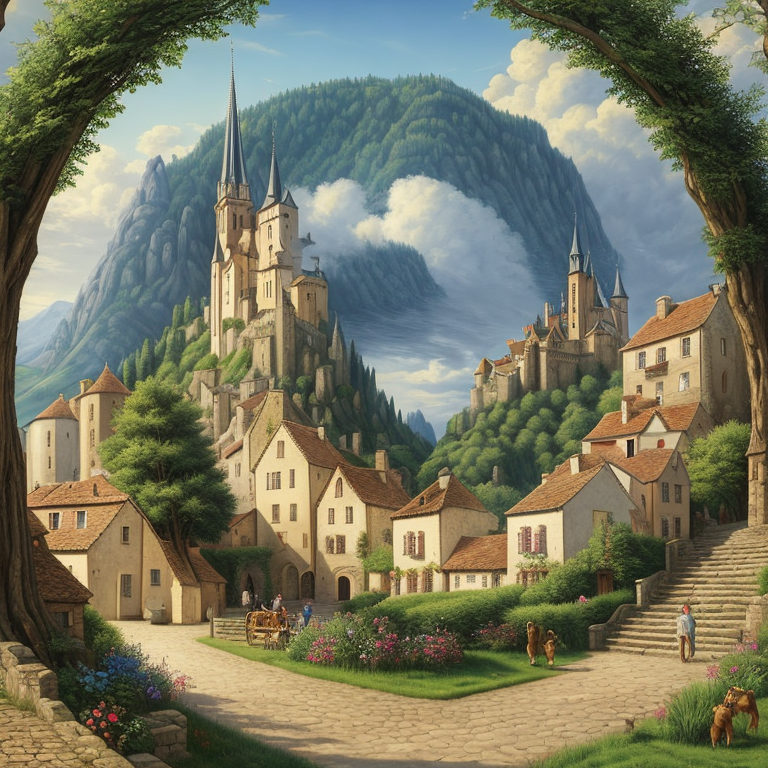
sd2.1-faceswapper
Take a picture/gif and replace the face in it with a face of your choice. You only need one image of the desired face. No dataset, no training
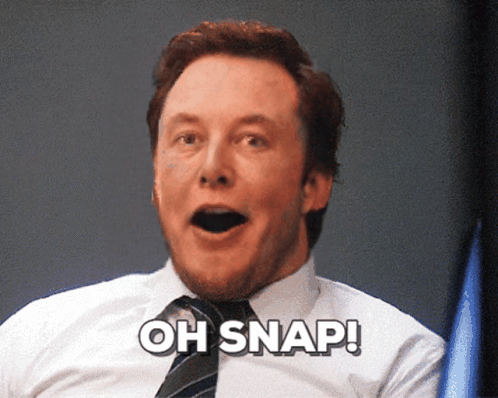