SAM v2 Image
SAM v2 Image (Segmentation Anything Model 2) represents a significant advancement in the field of computer vision, specifically addressing promptable visual segmentation in images. Developed by Meta AI, SAM v2 builds upon its predecessor, SAM, to provide robust and efficient solutions for segmenting objects in diverse visual contexts.
Model Information
-
Architecture: SAM v2 extends the original SAM model, incorporating innovations for image processing.
-
Promptable Segmentation: SAM v2 accepts various prompts (points, boxes, or masks) to identify and segment objects in images.
How to Use the Model
-
Upload an image (JPG, PNG, or WebP) using the provided field.
-
Coordinates for selecting objects in an image: Optionally, input coordinates (if needed). Provide either a prompt or coordinates. If a prompt is provided, coordinates will be ignored. For a single coordinate, use the format [834,74]. For multiple coordinates, use [[839,74], [844,20], ...].
-
Adjust advanced parameters:
-
Overlay Mask: Decide whether to overlay a mask on the image.
-
Remove Coordinates: If necessary, remove specific coordinates.
-
-
Click “Generate” to obtain the segmented image.
Use Cases
-
Assisted Image Labeling: SAM v2 assists in recommending annotations for images during labeling tasks.
-
Augmented Reality (AR) and Virtual Reality (VR): Accurate segmentation enhances realism in AR and VR applications.
-
Sonar Imaging: SAM v2 can be applied to underwater sonar images for object segmentation.
-
Autonomous Vehicles: Real-time segmentation aids in object detection for autonomous driving.
-
Environmental Monitoring: Use SAM v2 to segment objects in satellite imagery for environmental analysis.
Other Popular Models
fooocus
Fooocus enables high-quality image generation effortlessly, combining the best of Stable Diffusion and Midjourney.
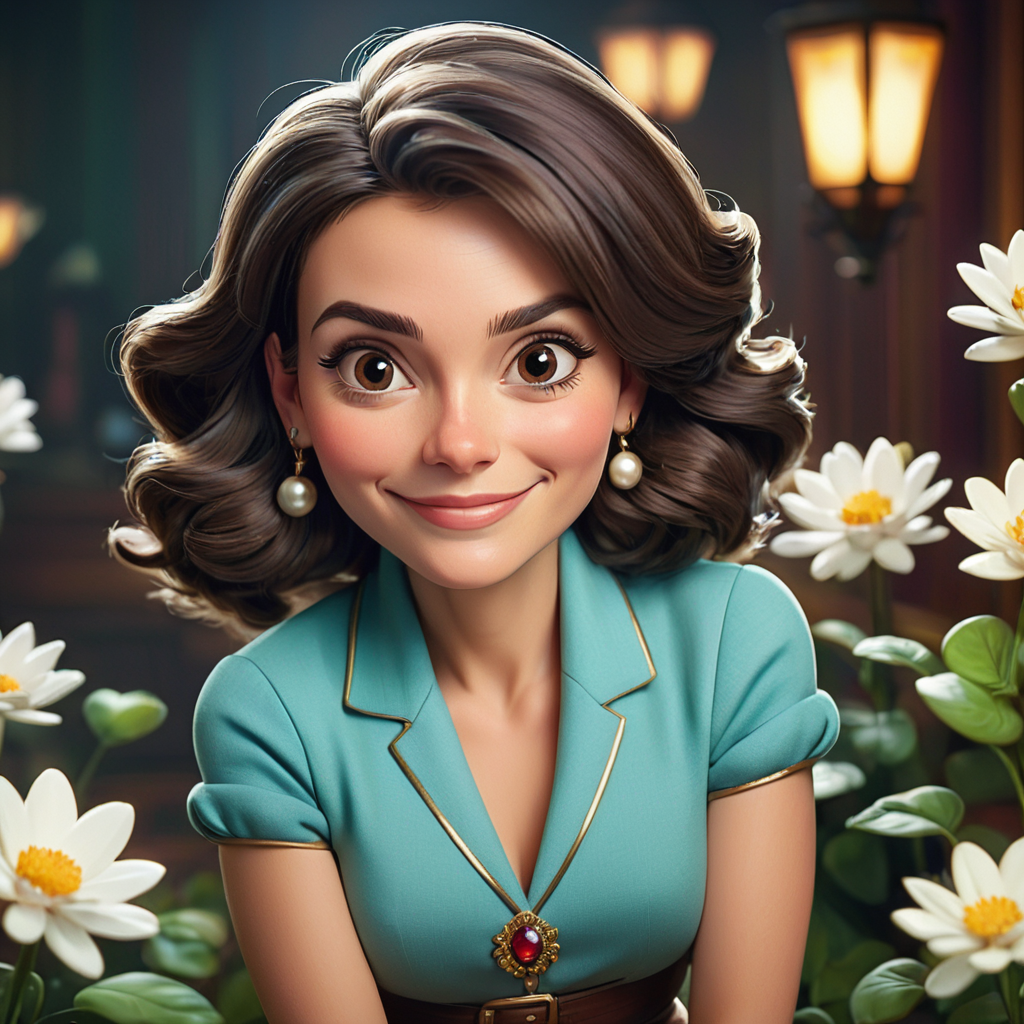
illusion-diffusion-hq
Monster Labs QrCode ControlNet on top of SD Realistic Vision v5.1
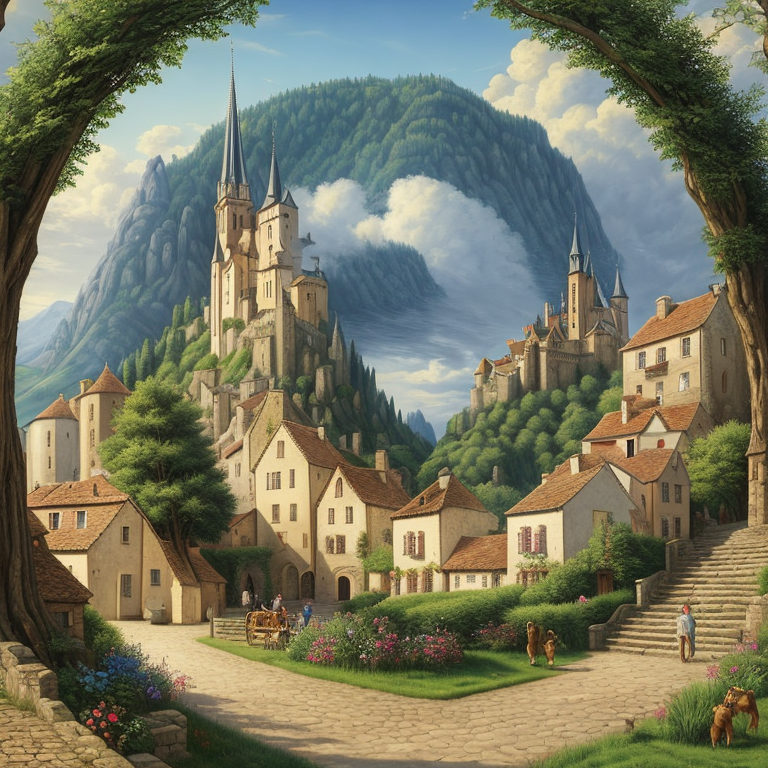
faceswap-v2
Take a picture/gif and replace the face in it with a face of your choice. You only need one image of the desired face. No dataset, no training
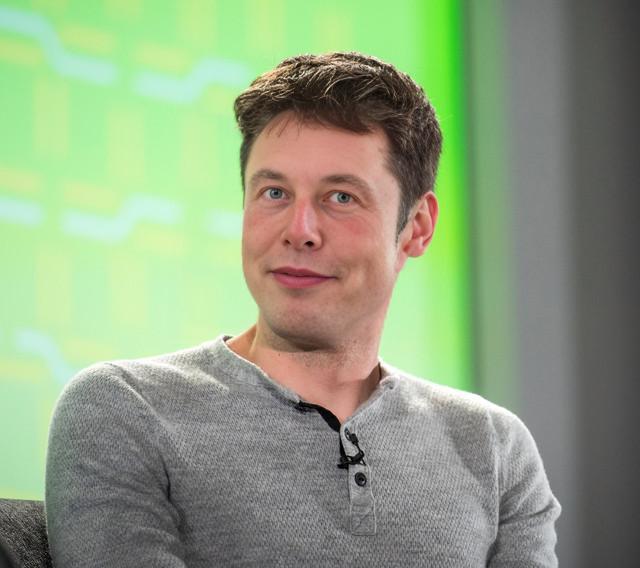
sd2.1-faceswapper
Take a picture/gif and replace the face in it with a face of your choice. You only need one image of the desired face. No dataset, no training
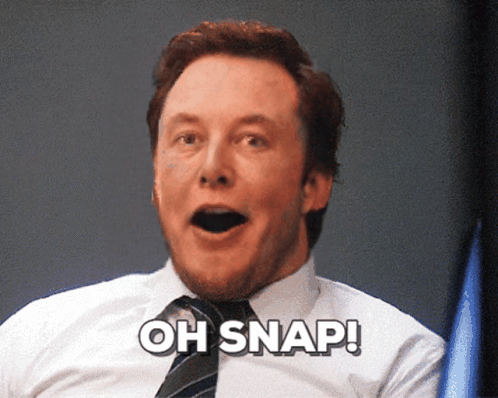