API
If you're looking for an API, you can choose from your desired programming language.
1
2
3
4
5
6
7
8
9
10
11
12
13
14
15
16
17
18
19
20
21
22
23
24
25
26
27
28
29
30
31
32
33
34
35
36
37
38
39
40
import requests
import base64
# Use this function to convert an image file from the filesystem to base64
def image_file_to_base64(image_path):
with open(image_path, 'rb') as f:
image_data = f.read()
return base64.b64encode(image_data).decode('utf-8')
# Use this function to fetch an image from a URL and convert it to base64
def image_url_to_base64(image_url):
response = requests.get(image_url)
image_data = response.content
return base64.b64encode(image_data).decode('utf-8')
# Use this function to convert a list of image URLs to base64
def image_urls_to_base64(image_urls):
return [image_url_to_base64(url) for url in image_urls]
api_key = "YOUR_API_KEY"
url = "https://api.segmind.com/v1/sd1.5-controlnet-canny"
# Request payload
data = {
"image": image_url_to_base64("https://segmind.com/canny-input.jpeg"), # Or use image_file_to_base64("IMAGE_PATH")
"samples": 1,
"prompt": "a colorful bird, 4k",
"negative_prompt": "Disfigured, cartoon, blurry, nude",
"scheduler": "UniPC",
"num_inference_steps": 25,
"guidance_scale": 7.5,
"strength": 1,
"seed": 3131738736286,
"base64": False
}
headers = {'x-api-key': api_key}
response = requests.post(url, json=data, headers=headers)
print(response.content) # The response is the generated image
Attributes
Input Image
Number of samples to generate.
min : 1,
max : 4
Prompt to render
Prompts to exclude, eg. 'bad anatomy, bad hands, missing fingers'
Type of scheduler.
Allowed values:
Number of denoising steps.
min : 20,
max : 100
Scale for classifier-free guidance
min : 0.1,
max : 25
How much to transform the reference image
min : 0.1,
max : 1
Seed for image generation.
Base64 encoding of the output image.
To keep track of your credit usage, you can inspect the response headers of each API call. The x-remaining-credits property will indicate the number of remaining credits in your account. Ensure you monitor this value to avoid any disruptions in your API usage.
ControlNet Canny
This cutting-edge model transcends traditional boundaries by employing the sophisticated canny edge detection method. Canny edge detection operates by pinpointing edges in an image through the identification of sudden shifts in intensity. Renowned for its prowess in accurately detecting edges while minimizing noise and erroneous edges, the method becomes even more potent when the preprocessor enhances its discernment by lowering the thresholds. Granting users an extraordinary level of control over image transformation parameters, the ControlNet Canny model is revolutionizing image editing and computer vision tasks, providing a customizable experience that caters to both subtle and dramatic image enhancements.
At the core of the ControlNet Canny model lies the renowned canny edge detection mechanism. This image-to-image transformation model is meticulously crafted to detect and emphasize edges in images. Its design allows users to tweak and adjust parameters, ensuring that the edge detection is tailored to specific needs, be it subtle enhancements or bold transformations.
Advantages
-
Precision Edge Detection: Utilizes the canny edge detection technique, known for its accuracy in identifying image edges.
-
User Control: Offers users the ability to fine-tune parameters, ensuring customized edge enhancements.
-
Versatility: Suitable for a myriad of applications, from artistic effects to technical image processing tasks.
-
Real-time Manipulation: Allows for instantaneous adjustments, providing users with real-time feedback and results.
##Use Cases
-
Image Segmentation: Ideal for tasks where precise edge identification is paramount, ensuring accurate segmentation.
-
Image Enhancement: Users can emphasize and highlight specific edges, enhancing the overall image quality and focus.
-
Artistic Effects: Artists and designers can leverage the model to create unique visual effects by manipulating image edges.
-
Advanced Image Editing Software: Can be integrated as a feature, offering users advanced edge manipulation capabilities.
-
Computer Vision Tasks: Perfect for algorithms that require accurate edge detection for object recognition or feature extraction.
ControlNet Canny License
ControlNet Canny, in its commitment to ethical AI practices, has embraced the CreativeML OpenRAIL M license. This decision not only underscores the model's dedication to responsible AI but also aligns it with the principles set forth by BigScience and the RAIL Initiative. Their collaborative work in AI ethics and responsibility has set the benchmark for licenses like the OpenRAIL M.
Other Popular Models
sdxl-img2img
SDXL Img2Img is used for text-guided image-to-image translation. This model uses the weights from Stable Diffusion to generate new images from an input image using StableDiffusionImg2ImgPipeline from diffusers
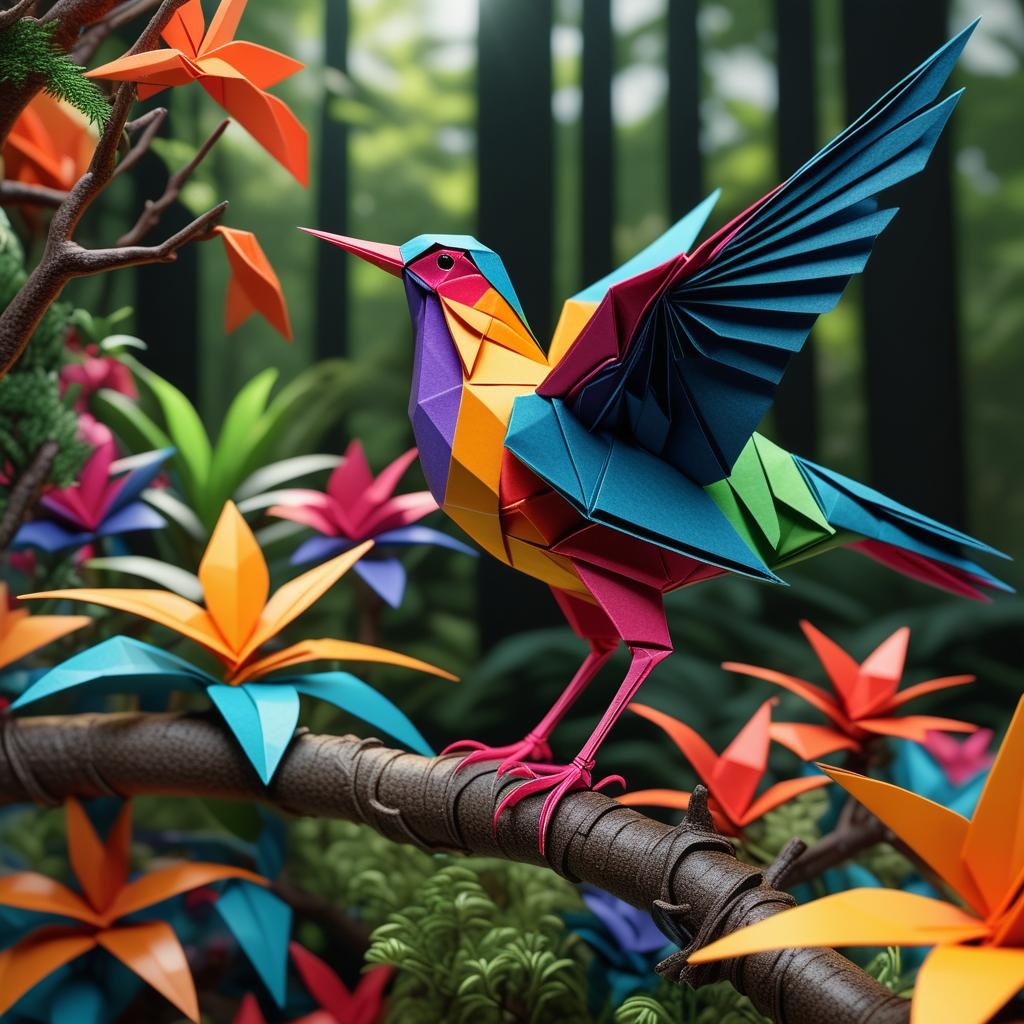
fooocus
Fooocus enables high-quality image generation effortlessly, combining the best of Stable Diffusion and Midjourney.
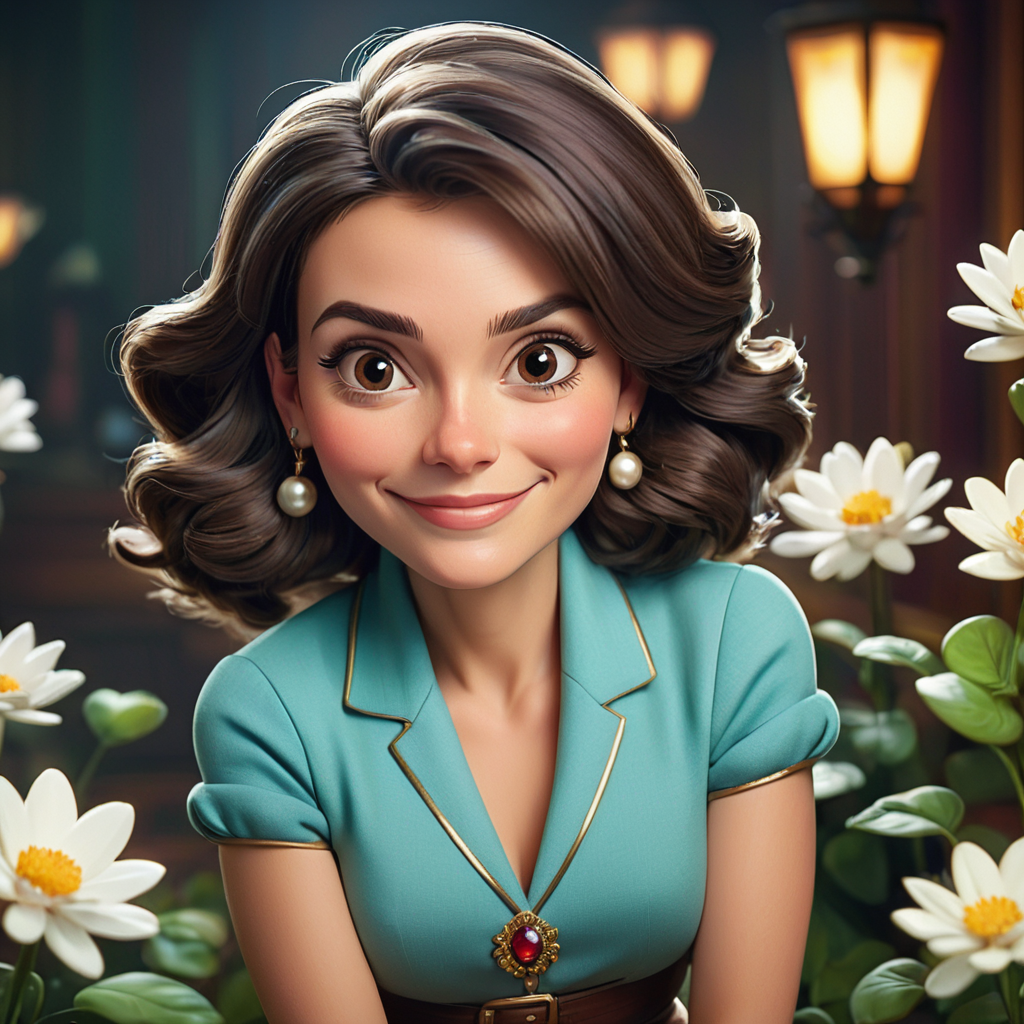
sdxl1.0-txt2img
The SDXL model is the official upgrade to the v1.5 model. The model is released as open-source software
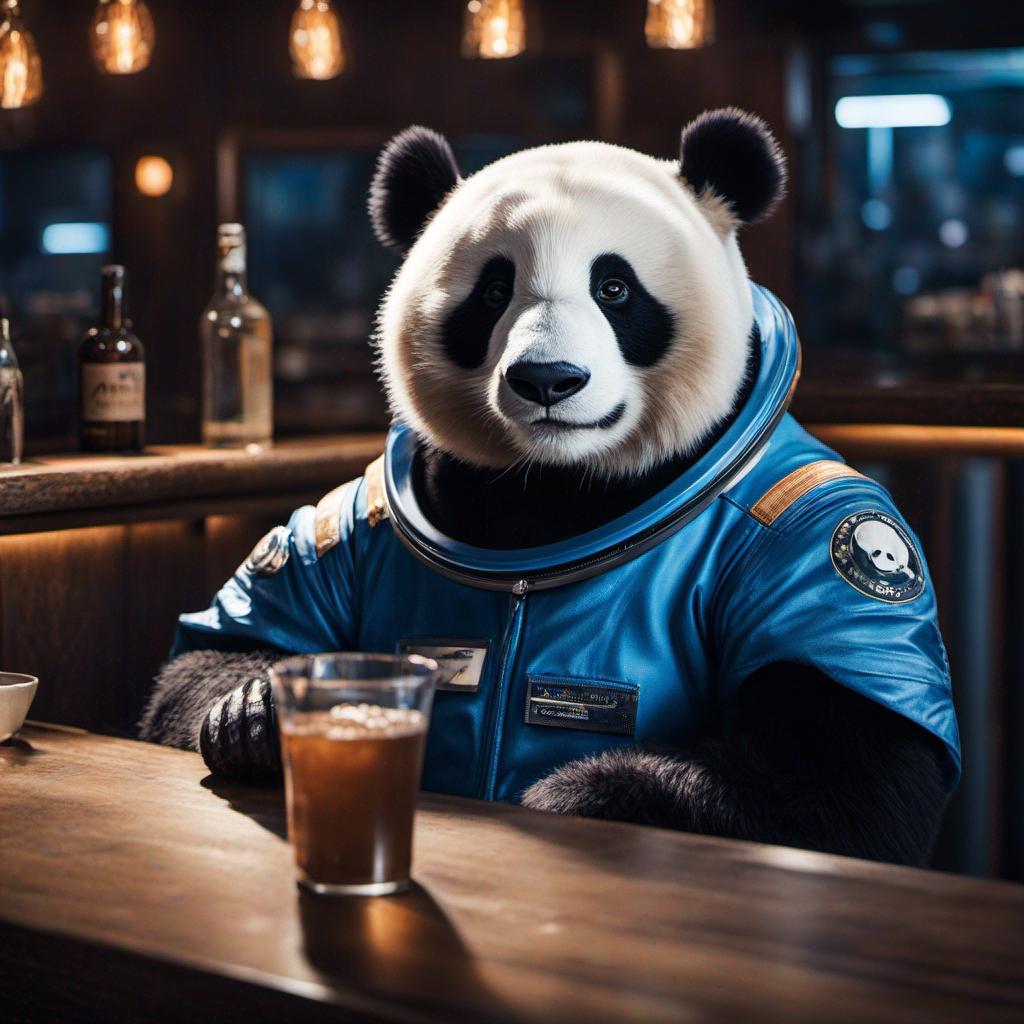
codeformer
CodeFormer is a robust face restoration algorithm for old photos or AI-generated faces.
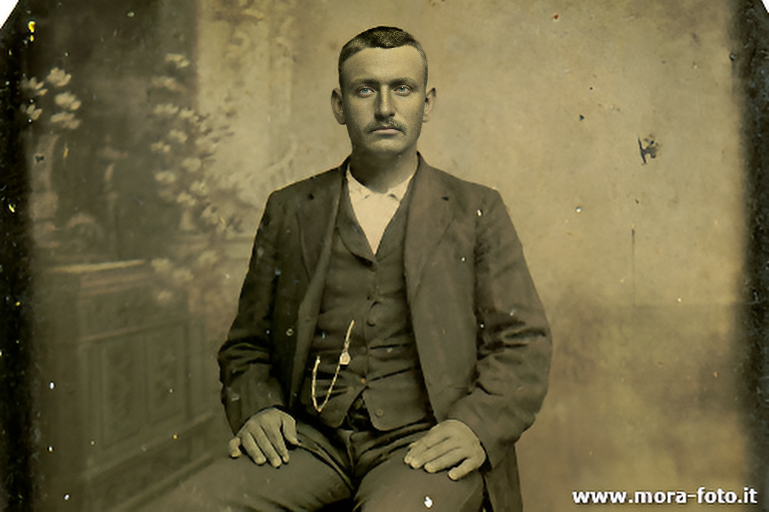