API
If you're looking for an API, you can choose from your desired programming language.
1
2
3
4
5
6
7
8
9
10
11
12
13
14
15
16
17
18
19
20
21
22
23
24
25
26
27
import requests
import base64
# Use this function to convert an image file from the filesystem to base64
def image_file_to_base64(image_path):
with open(image_path, 'rb') as f:
image_data = f.read()
return base64.b64encode(image_data).decode('utf-8')
# Use this function to fetch an image from a URL and convert it to base64
def image_url_to_base64(image_url):
response = requests.get(image_url)
image_data = response.content
return base64.b64encode(image_data).decode('utf-8')
api_key = "YOUR_API_KEY"
url = "https://api.segmind.com/v1/sam-img2img"
# Request payload
data = {
"image": image_url_to_base64("https://segmind.com/kitchen.jpg") # Or use image_file_to_base64("IMAGE_PATH")
}
headers = {'x-api-key': api_key}
response = requests.post(url, json=data, headers=headers)
print(response.content) # The response is the generated image
Attributes
Image to Segment
To keep track of your credit usage, you can inspect the response headers of each API call. The x-remaining-credits property will indicate the number of remaining credits in your account. Ensure you monitor this value to avoid any disruptions in your API usage.
Segment Anything Model (SAM)
The Segment Anything Model (SAM) is the latest groundbreaking AI model introduced by Meta AI. With the power to "cut out" any object from any image with just a single click, SAM is revolutionizing the world of image processing. Zero-shot capability; Unlike other models that require extensive training for each new object or image, SAM boasts of a promptable segmentation system. This means it can easily generalize to unfamiliar objects and images without the need for any additional training.
The SAM model architecture integrates a masked auto-encoder (MAE) and a Vision Transformer (ViT) to generate distinct image embeddings, typically initialized before model prompting. SAM's prompt encoding component transforms elements such as points, masks, and texts into immediate vectors, categorizing prompts into sparse or dense groups. Subsequently, SAM's mask decoder predicts segmentation masks by leveraging these embeddings, aligning them with a specific output token. Through bidirectional cross-attention, the labeled masks are processed to enhance SAM's weights, ensuring its adaptability and streamlined performance.
Advantages
-
Zero-shot Learning: SAM can identify and segment unfamiliar objects without any prior training, making it highly versatile.
-
Promptable System: Users can easily guide SAM using prompts, ensuring more accurate results tailored to specific needs.
-
Speed and Efficiency: Thanks to its advanced architecture, SAM processes images rapidly, delivering results in real-time.
-
High Precision : SAM's extensive training and hybrid architecture ensure that the segmented results are of the highest quality.
Use cases
-
E-commerce Product Listing: Retailers can use SAM to automatically segment product images, removing backgrounds and placing products on a consistent backdrop.
-
Photo Editing Apps: SAM can be integrated into photo editing software, allowing users to easily extract objects and create composite images.
-
Augmented Reality (AR) Applications: SAM can help in real-time object segmentation, enabling developers to overlay virtual objects onto real-world scenes seamlessly.
-
Medical Imaging: Healthcare professionals can use SAM to segment specific organs or anomalies in medical images, aiding in diagnosis and treatment planning.
-
Video Production: Film and video editors can utilize SAM to segment and replace backgrounds, create special effects, or integrate CGI elements into real footage.
SAM License
The Segment Anything Model (SAM) is open source. Meta AI has released the SA-1B Dataset for research purposes and also made available the pre-trained models (approximately 2.4 GB) and associated code. All of these are licensed under the Apache 2.0, a permissive license, in line with FAIR's dedication to open research.
Other Popular Models
sdxl-img2img
SDXL Img2Img is used for text-guided image-to-image translation. This model uses the weights from Stable Diffusion to generate new images from an input image using StableDiffusionImg2ImgPipeline from diffusers
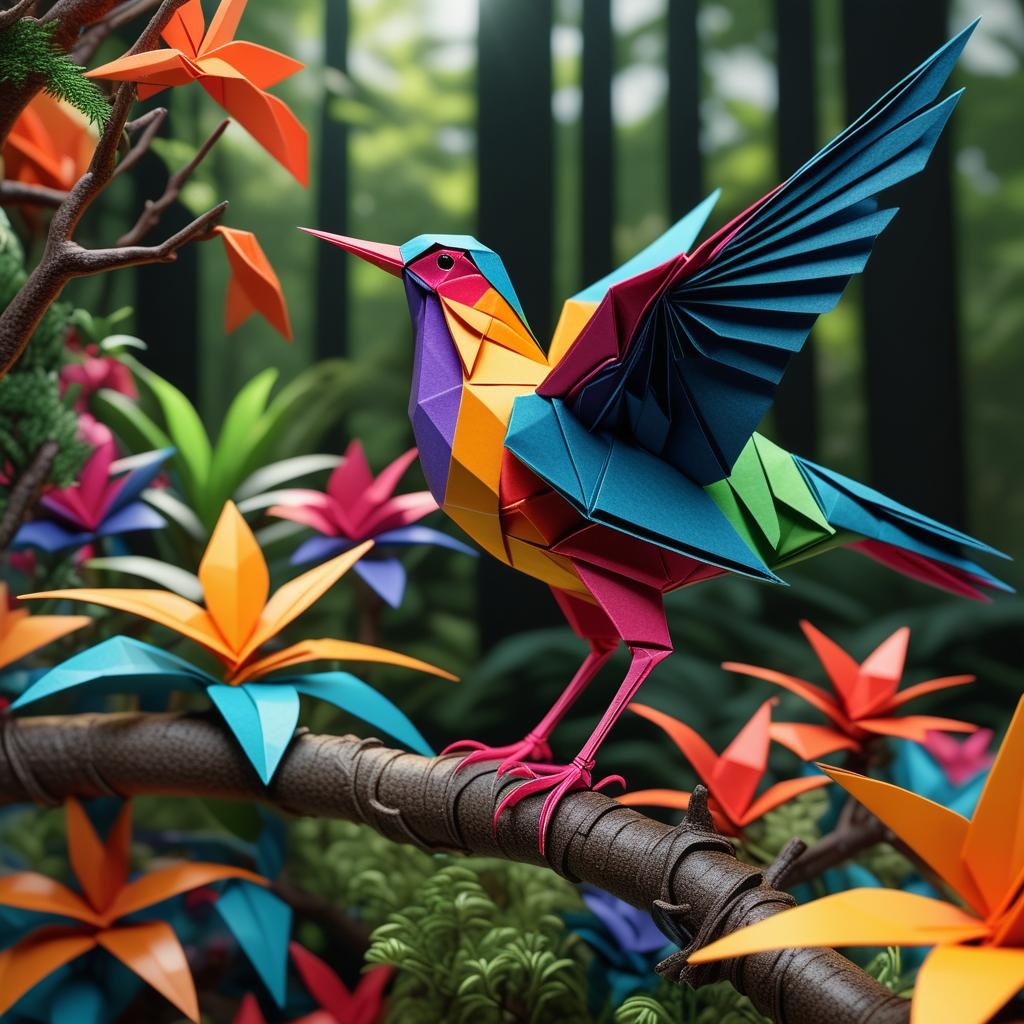
face-to-many
Turn a face into 3D, emoji, pixel art, video game, claymation or toy
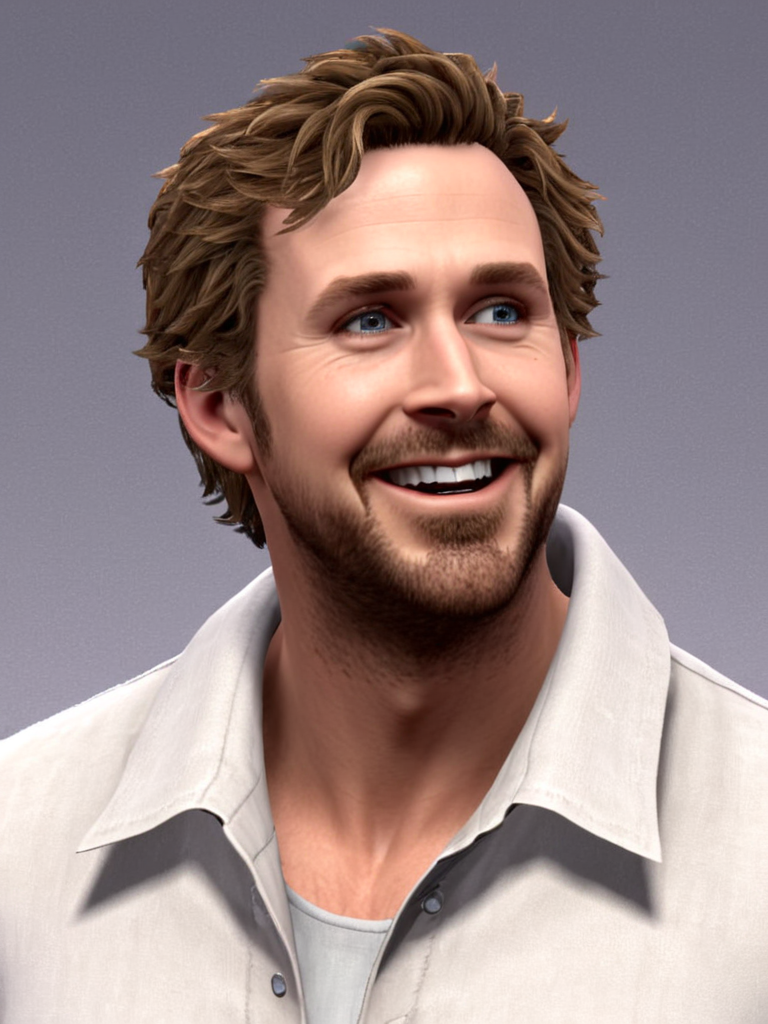
sdxl1.0-txt2img
The SDXL model is the official upgrade to the v1.5 model. The model is released as open-source software
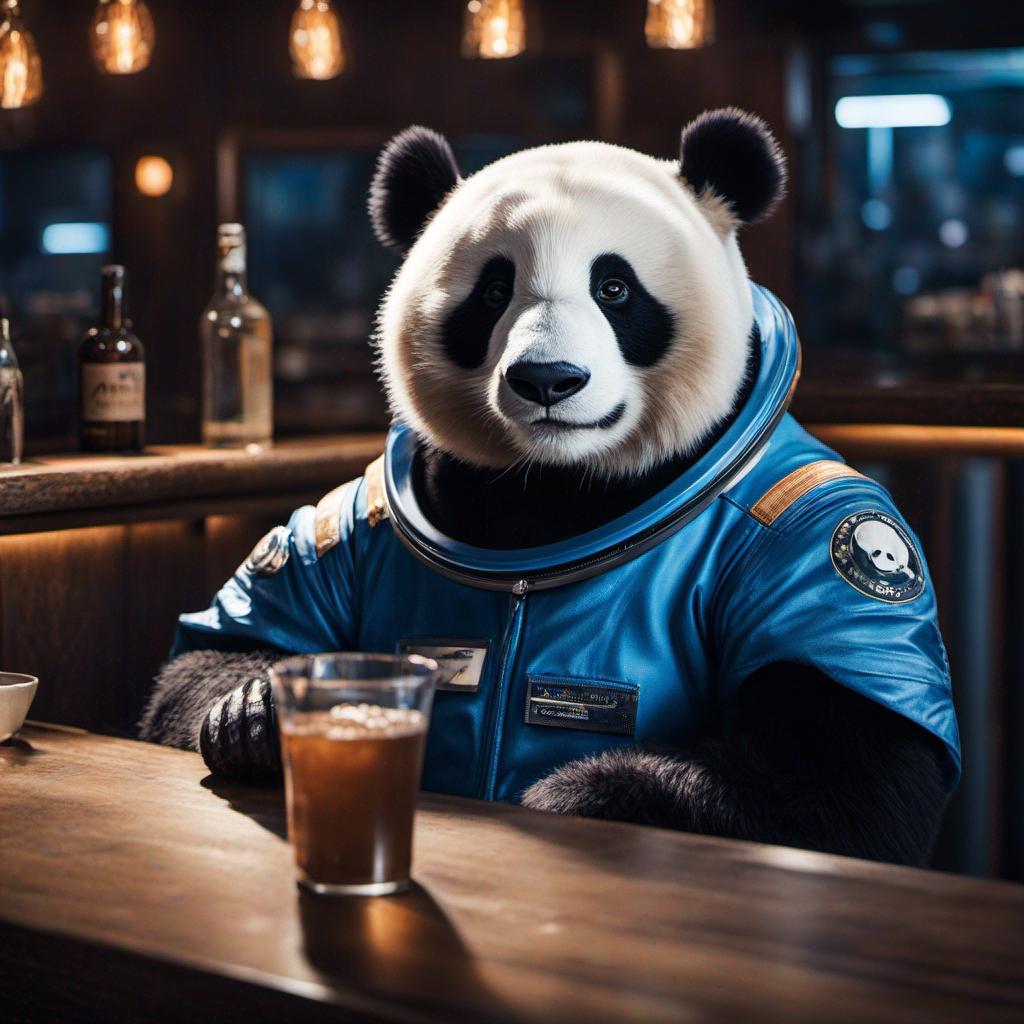
sd2.1-faceswapper
Take a picture/gif and replace the face in it with a face of your choice. You only need one image of the desired face. No dataset, no training
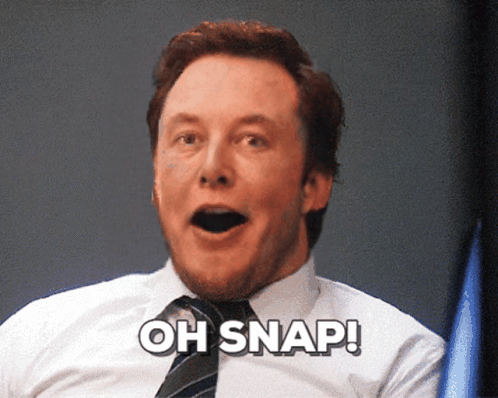