Text Embedding 3 Small
Text-embedding-3-small is a compact and efficient model developed for generating high-quality text embeddings. These embeddings are numerical representations of text data, enabling a variety of natural language processing (NLP) tasks such as semantic search, clustering, and text classification
API
If you're looking for an API, you can choose from your desired programming language.
1
2
3
4
5
6
7
8
9
10
11
12
13
14
15
16
17
18
19
20
21
22
23
24
25
26
27
import requests
import base64
# Use this function to convert an image file from the filesystem to base64
def image_file_to_base64(image_path):
with open(image_path, 'rb') as f:
image_data = f.read()
return base64.b64encode(image_data).decode('utf-8')
# Use this function to fetch an image from a URL and convert it to base64
def image_url_to_base64(image_url):
response = requests.get(image_url)
image_data = response.content
return base64.b64encode(image_data).decode('utf-8')
api_key = "YOUR_API_KEY"
url = "https://api.segmind.com/v1/text-embedding-3-small"
# Request payload
data = {
"prompt": "You are beautiful"
}
headers = {'x-api-key': api_key}
response = requests.post(url, json=data, headers=headers)
print(response.content) # The response is the generated image
Attributes
Prompt to render
To keep track of your credit usage, you can inspect the response headers of each API call. The x-remaining-credits property will indicate the number of remaining credits in your account. Ensure you monitor this value to avoid any disruptions in your API usage.
Text Embedding 3 Small
Text-embedding-3-small is a compact and efficient model developed for generating high-quality text embeddings. These embeddings are numerical representations of text data, enabling a variety of natural language processing (NLP) tasks such as semantic search, clustering, and text classification. The smaller model size allows for faster processing and lower resource consumption while maintaining robust performance.
How to Fine-Tune Outputs?
Input Text Length: Balance text length according to the specific task requirements. Short texts may not capture enough context, while very long texts might need truncation or summarization strategies.
Use Cases
Text-embedding-3-small is versatile and can be deployed in numerous NLP applications:
-
Semantic Search: Enhance search engines by leveraging embeddings to measure similarity between user queries and documents.
-
Text Classification: Use embeddings as input features for training machine learning models in various classification tasks.
-
Clustering and Topic Modeling: Employ clustering algorithms on embeddings to identify topics or group similar texts in a corpus.
-
Recommendation Systems: Improve recommendation accuracy by computing and comparing embeddings of user queries and item descriptions.
Other Popular Models
sdxl-controlnet
SDXL ControlNet gives unprecedented control over text-to-image generation. SDXL ControlNet models Introduces the concept of conditioning inputs, which provide additional information to guide the image generation process
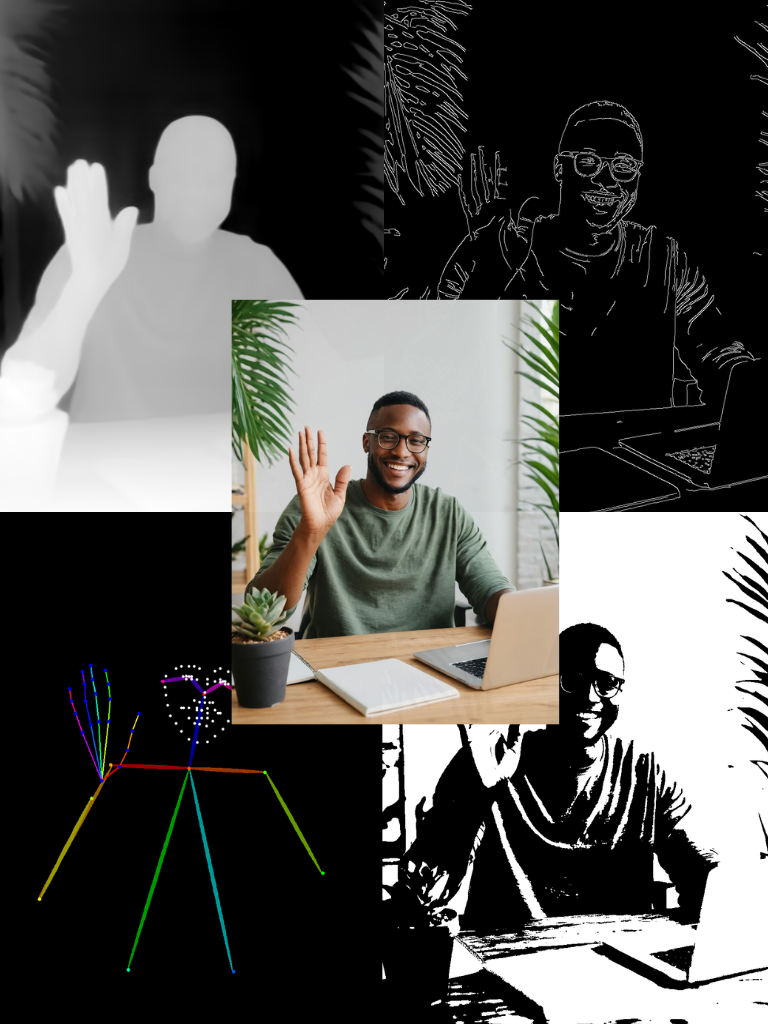
storydiffusion
Story Diffusion turns your written narratives into stunning image sequences.
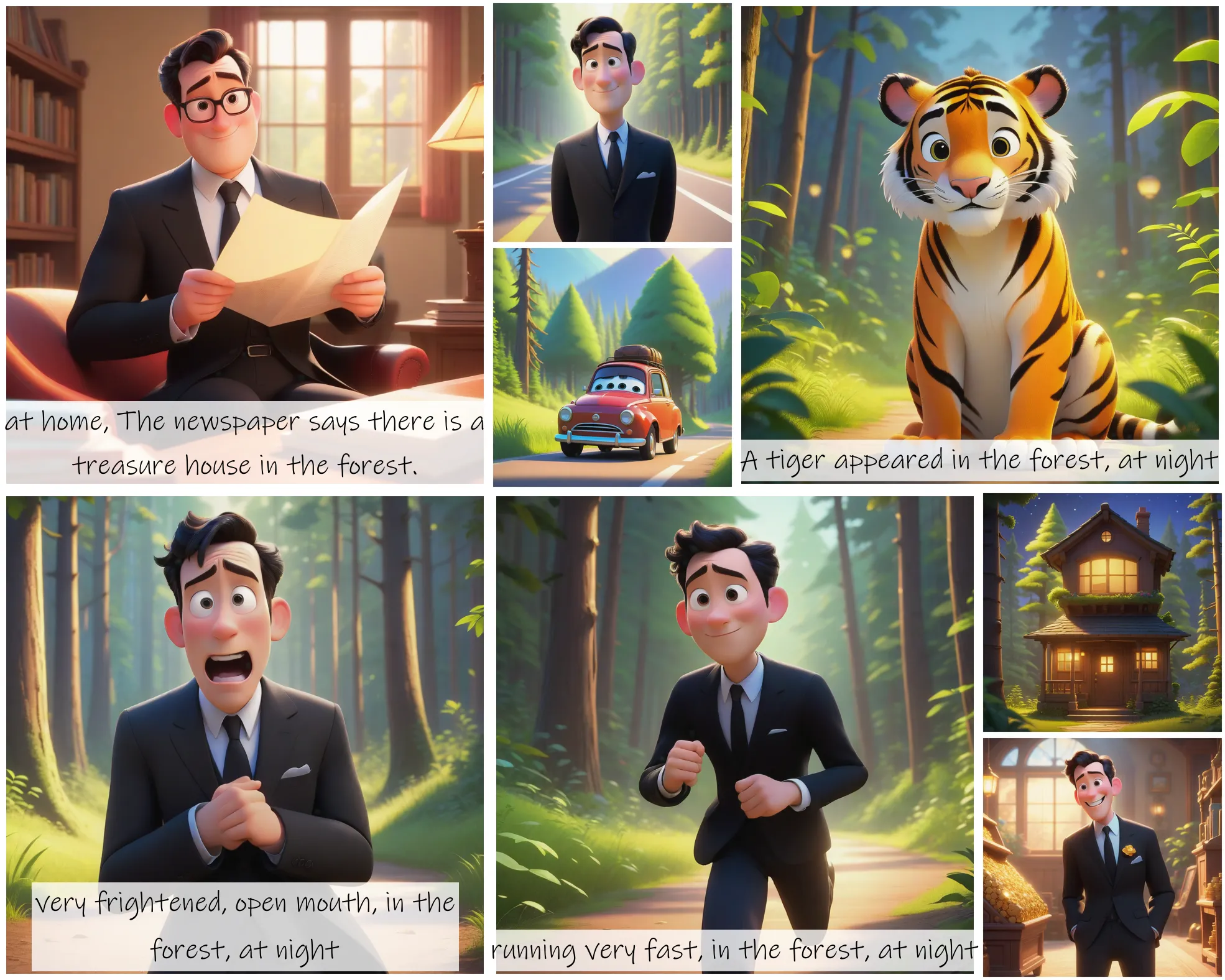
codeformer
CodeFormer is a robust face restoration algorithm for old photos or AI-generated faces.
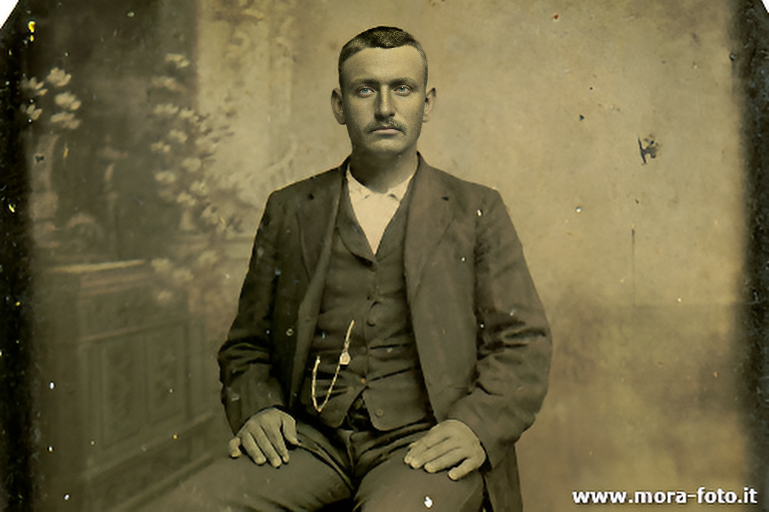
sd2.1-faceswapper
Take a picture/gif and replace the face in it with a face of your choice. You only need one image of the desired face. No dataset, no training
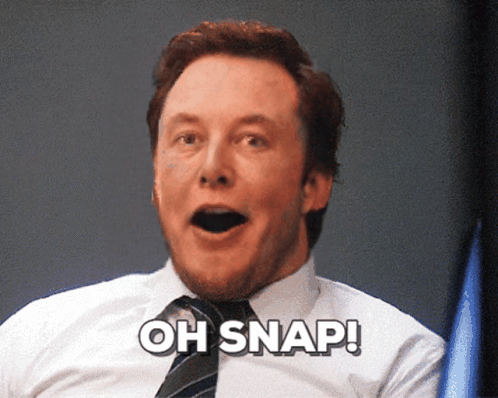